User login
A simple algorithm for predicting bacteremia using food consumption and shaking chills: a prospective observational study
Fever in hospitalized patients is a nonspecific finding with many potential causes. Blood cultures (BC) are commonly obtained prior to commencing parenteral antibiotics in febrile patients. However, as many as 35% to 50% of positive BCs represent a contamination with organisms inoculated from the skin into culture bottles at the time of sample collection.1-3 Such results represent false-positive BCs that can lead to unnecessary investigations and treatment.
Recently, Coburn et al. reviewed the severity of chills (graded on an ordinal scale) as the most useful predictor of true bacteremia (positive likelihood ratio [LR], 4.7; 95% confidence interval [CI], 3.0–7.2),4-6 and the lack of the systemic inflammatory response syndrome (SIRS) criteria as the best negative indicator of true bacteremia with a negative LR of 0.09 (95% CI, 0.03-0.3).6,7 We have also previously reported normal food consumption as a negative indicator of true bacteremia, with a 98.3% negative predictive value.8 Henderson’s Basic Principles of Nursing Care emphasizes the importance of evaluating whether a patient can eat and drink adequately,9 and the evaluation of a patient’s food consumption is a routine nursing staff practice, which is treated as vital sign in Japan, in contrast to nursing practices in the United States.
However, these data were the result of a single-center retrospective study using the nursing staff’s assessment of food consumption, and they cannot be generalized to larger patient populations. Therefore, the aim of this prospective, multicenter study was to measure the accuracy of food consumption and shaking chills as predictive factors for true bacteremia.
METHODS
Study Design
This was a prospective multicenter observational study (UMIN ID: R000013768) involving 3 hospitals in Tokyo, Japan, that enrolled consecutive patients who had BCs obtained. This study was approved by the ethical committee at Juntendo University Nerima Hospital and each of the participating centers, and the study was conducted in accordance with the Declaration of Helsinki 1971, as revised in 1983. We evaluated 2,792 consecutive hospitalized patients (mean age, 68.9 ± 17.1 years; 55.3% men) who had BCs obtained between April 2013 and August 2014, inclusive. The indication for BC acquisition was at the discretion of the treating physician. The study protocol and the indication for BCs are described in detail elsewhere.8 We excluded patients with anorexia-inducing conditions such as gastrointestinal disease, including gastrointestinal bleeding, enterocolitis, gastric ulceration, peritonitis, appendicitis, cholangitis, pancreatitis, diverticulitis, and ischemic colitis. We also excluded patients receiving chemotherapy for malignancy. In this study, true bacteremia was defined as identical organisms isolated from 2 sets of blood cultures (a set refers to one aerobic bottle and one anaerobic bottle). Moreover, even if only one set of blood cultures was acquired, when the identified pathogen could account for the clinical presentation, we also defined this as true bacteremia. Briefly, contaminants were defined as organisms common to skin flora, including Bacillus species, coagulase-negative Staphylococcus, Corynebacterium species, and Micrococcus species, without isolation of an identical organism with the same antibiotic susceptibilities from another potentially infected site in a patient with incompatible clinical features and no risk factors for infection with the isolated organism. Single BCs that were positive for organisms that were unlikely to explain the patient’s symptoms were also considered as contaminants. Patients with contaminated BCs were excluded from the analyses.
Structure of Reliability Study Procedures
Nurses in the 3 different hospitals performed daily independent food consumption ratings during each patient’s stay. Interrater reliability assessments were conducted in the morning or afternoon, and none of the raters had access to the other nurses’ scores at any time. The study nurses performed simultaneous ratings during these assessments (one interacted with and rated the patient while the other observed and rated the same patient).
Prediction Variables of True Bacteremia
1. Food consumption. Assessment of food consumption has been previously described in detail.8 Briefly, we characterized the patients’ oral intake based on the meal taken immediately prior to the BCs. For example, if a fever developed at 2
2. Chills. As done previously, the physician evaluated the patient for a history of chills at the time of BCs and classified the patients into 1 of 4 grades4,5: “no chills,” the absence of any chills; “mild chills,” feeling cold, equivalent to needing an outer jacket; “moderate chills,” feeling very cold, equivalent to needing a thick blanket; and “shaking chills,” feeling extremely cold with rigors and generalized bodily shaking, even under a thick blanket. To distinguish between those patients who had shaking chills and those who did not, we divided the patients into 2 groups: the “shaking chills group” and the combination of none, mild, and moderate chills, referred to as the “negative shaking chills group.”
3. Other predictive variables. We considered the following additional predictive variables: age, gender, axillary body temperature (BT), heart rate (HR), systolic blood pressure (SBP), respiratory rate (RR), white blood cell count (WBC), and serum C-reactive protein level (CRP). These predictive variables were obtained immediately prior to the BCs. We defined SIRS based on standard criteria (HR >90 beats/m, RR >20/m, BT <36°C or >38°C, and a WBC <4 × 103 WBC/μL or >12 × 103 WBC/μL). Patients were subcategorized by age into 2 groups (≤69 years and >70 years). CRP levels were dichotomized as >10.0 mg/dL or ≤10.0 mg/dL. We reviewed the patients’ charts to determine whether they had received antibiotics. In the case of walk-in patients, we interviewed the patients regarding whether they had visited a clinic; if they had, they were questioned as to whether any antibiotic therapy had been prescribed.
Statistical Analysis
Continuous variables are presented as the mean with the associated standard deviation (SD). All potential variables predictive of true bacteremia are shown in Table 1. The variables were dichotomized by clinically meaningful thresholds and used as potential risk-adjusted variables. We calculated the sensitivity and specificity and positive and negative predictive value for each criterion. Multiple logistic regression analysis was used to select components that were significantly associated with true bacteremia (the level of statistical significance determined with maximum likelihood methods was set at P < .05). To visualize and quantify other aspects in the prediction of true bacteremia, a recursive partitioning analysis (RPA) was used to make a decision tree model for true bacteremia. This nonparametric regression method produces a classification tree following a series of nonsequential top-down binary splits. The tree-building process starts by considering a set of predictive variables and selects the variable that produces 2 subsets of participants with the greatest purity. Two factors are considered when splitting a node into its daughter nodes: the goodness of the split and the amount of impurity in the daughter nodes. The splitting process is repeated until further partitioning is no longer possible and the terminal nodes have been reached. Details on this method are discussed in Monte Carlo Calibration of Distributions of Partition Statistics (www.jmp.com).
Probability was considered significant at a value of P < .05. All statistical tests were 2-tailed. Statistical analyses were conducted by a physician (KI) and an independent statistician (JM) with the use of the SPSS® v.16.0 software package (SPSS Inc., Chicago, IL) and JMP® version 8.0.2 (SAS Institute, Cary, NC).
RESULTS
Patients Characteristics
Two thousand seven hundred and ninety-two patients met the inclusion criteria for our study, from which 849 were excluded (see Figure 1 for flow diagram). Among the remaining 1,943 patients, there were 317 patients with positive BCs, of which 221 patients (69.7%) were considered to have true-positive BCs and 96 (30.3%) were considered to have contaminated BCs. After excluding these 96 patients, 221 patients with true bacteremia (true bacteremic group) were compared with 1,626 nonbacteremic patients (nonbacteremic group; Figure 1). The baseline characteristics of the subjects are shown in Table 1. The mean BT was 38.4 ± 1.2°C in the true bacteremic group and 37.9 ± 1.0°C in the nonbacteremic group. The mean serum CRP level was 11.6 ± 9.6 mg/dL in the true bacteremic group and 7.3 ± 6.9 mg/dL in the nonbacteremic group. In the true bacteremic group, there were 6 afebrile patients, and 27 patients without leukocytosis. The pathogens identified from the true-positive BCs were Escherichia coli (n = 59, 26.7%), including extended-spectrum beta-lactamase producing species, Staphylococcus aureus (n = 36, 16.3%), including methicillin-resistant Staphylococcus aureus, and Klebsiella pneumoniae (n = 22, 10.0%; Supplemental Table 1).
The underlying clinical diagnoses in the true bacteremic group included urinary tract infection (UTI), pneumonia, abscess, catheter-related bloodstream infection (CRBSI), cellulitis, osteomyelitis, infective endocarditis (IE), chorioamnionitis, iatrogenic infection at hemodialysis puncture sites, bacterial meningitis, septic arthritis, and infection of unknown cause (Supplemental Table 2).
Interrater Reliability Testing of Food Consumption
Patients were evaluated during their hospital stays. The interrater reliability of the evaluation of food consumption was very high across all participating hospitals (Supplemental Table 3). To assess the reliability of the evaluations of food consumption, patients (separate from this main study) were selected randomly and evaluated independently by 2 nurses in 3 different hospitals. The kappa scores of agreement between the nurses at the 3 different hospitals were 0.83 (95% CI, 0.63-0.88), 0.90 (95% CI, 0.80-0.99), and 0.80 (95% CI, 0.67-0.99), respectively. The interrater reliability of food consumption evaluation by the nurses was very high at all participating hospitals.
Food Consumption
The low, moderate, and high food consumption groups consisted of 964 (52.1%), 306 (16.6%), and 577 (31.2%) patients, respectively (Table 1). Of these, 174 (18.0%), 33 (10.8%), and 14 (2.4%) patients, respectively, had true bacteremia. The presence of poor food consumption had a sensitivity of 93.7% (95% CI, 89.4%-97.9%), specificity of 34.6% (95% CI, 33.0%-36.2%), and a positive LR of 1.43 (95% CI, 1.37-1.50) for predicting true bacteremia. Conversely, the absence of poor food consumption (ie, normal food consumption) had a negative LR of 0.18 (95% CI, 0.17-0.19).
Chills
The no, mild, moderate, and shaking chills groups consisted of 1,514 (82.0%), 148 (8.0%), 53 (2.9%), and 132 (7.1%) patients, respectively (Table 1). Of these, 136 (9.0%), 25 (16.9%), 8 (15.1%), and 52 (39.4%) patients, respectively, had true bacteremia. The presence of shaking chills had a sensitivity of 23.5% (95% CI, 22.5%-24.6%), a specificity of 95.1% (95% CI, 90.7%-99.4%), and a positive LR of 4.78 (95% CI, 4.56–5.00) for predicting true bacteremia. Conversely, the absence of shaking chills had a negative LR of 0.80 (95% CI, 0.77-0.84).
Prediction Model for True Bacteremia
The components identified as significantly related to true bacteremia by multiple logistic regression analysis are indicated in Table 2. The significant predictors of true bacteremia were shaking chills (odds ratio [OR], 5.6; 95% CI, 3.6-8.6; P < .01), SBP <90 mmHg (OR, 3.1; 95% CI, 1.6-5.7; P < 01), CRP levels >10.0 mg/dL (OR, 2.2; 95% CI, 1.6-3.1; P < .01), BT <36°C or >38°C (OR, 1.8; 95% CI, 1.3-2.6; P < .01), WBC <4 × 103/μL or >12 × 103/μL (OR, 1.6; 95% CI, 1.2-2.3; P = .003), HR >90 bpm (OR, 1.5; 95% CI, 1.1-2.1; P = .021), and female (OR, 1.4; 95% CI, 1.0-1.9; P = .036). An RPA to create an ideal prediction model for patients with true bacteremia or nonbacteremia is shown in Figure 2. The original group consisted of 1,847 patients, including 221 patients with true bacteremia. The pretest probability of true bacteremia was 2.4% (14/577) for those with normal food consumption (Group 1) and 2.4% (13/552) for those with both normal food consumption and the absence of shaking chills (Group 2). Conversely, the pretest probability of true bacteremia was 16.3% (207/1270) for those with poor food consumption and 47.7% (51/107) for those with both poor food consumption and shaking chills. The patients with true bacteremia with normal food consumption and without shaking chills consisted of 4 cases of CRBSI and UTI, 2 cases of osteomyelitis, 1 case of IE, 1 case of chorioamnionitis, and 1 case for which the focus was unknown (Supplemental Table 4).
DISCUSSION
In this observational study, we evaluated if a simple algorithm using food consumption and shaking chills was useful for assessing whether a patient had true bacteremia. A 2-item screening checklist (nursing assessment of food consumption and shaking chills) had excellent statistical properties as a brief screening instrument for true bacteremia.
We have prospectively validated that food consumption, as assessed by nurses, is a reliable predictor of true bacteremia.8 A previous single-center retrospective study showed similar findings, but these could not be generalized across all institutions because of the limited nature of the study. In this multicenter study, we used 2 statistical methods to reduce selection bias. First, we performed a kappa analysis across the hospitals to evaluate the interrater reliability of the evaluation of food consumption. Second, we used an RPA (Figure 2), also known as a decision tree model. RPA is a step-by-step process by which a decision tree is constructed by either splitting or not splitting each node on the tree into 2 daughter nodes.10 By using this method, we successfully generated an ideal approach to predict true bacteremia using food consumption and shaking chills. After adjusting for food consumption and shaking chills, groups 1 to 2 had sequentially decreasing diagnoses of true bacteremia, varying from 221 patients to only 13 patients.
Appetite is influenced by many factors that are integrated by the brain, most importantly within the hypothalamus. Signals that impinge on the hypothalamic center include neural afferents, hormones, cytokines, and metabolites.11 These factors elicit “sickness behavior,” which includes a decrease in food-motivated behavior.12 Furthermore, exposure to pathogenic bacteria increases serotonin, which has been shown to decrease metabolism in
The strengths of this study include its relatively large sample size, multicenter design, uniformity of data collection across sites, and completeness of data collection from study participants. All of these factors allowed for a robust analysis.
However, there are several limitations of this study. First, the physicians or nurses asked the patients about the presence of shaking chills when they obtained the BCs. It may be difficult for patients, especially elderly patients, to provide this information promptly and accurately. Some patients did not call the nurse when they had shaking chills, and the chills were not witnessed by a healthcare provider. However, we used a more specific definition for shaking chills: a feeling of being extremely cold with rigors and generalized bodily shaking, even under a thick blanket. Second, this algorithm is not applicable to patients with immunosuppressed states because none of the hospitals involved in this study perform bone marrow or organ transplantation. Third, although we included patients with dementia in our cohort, we did not specifically evaluate performance of the algorithm in patients with this medical condition. It is possible that the algorithm would not perform well in this subset of patients owing to their unreliable appetite and food intake. Fourth, some medications may affect appetite, leading to reduced food consumption. Although we have not considered the details of medications in this study, we found that the pretest probability of true bacteremia was low for those patients with normal food consumption regardless of whether the medication affected their appetites or not. However, the question of whether medications truly affect patients’ appetites concurrently with bacteremia would need to be specifically addressed in a future study.
CONCLUSION
In conclusion, we have established a simple algorithm to identify patients with suspected true bacteremia who require the acquisition of blood cultures. This extremely simple model can enable physicians to make a rapid bedside estimation of the risk of true bacteremia.
Acknowledgment
The authors thank Drs. H. Honda and S. Saint, and Ms. A. Okada for their helpful discussions with regard to this study; Ms. M. Takigawa for the collection of data; and Ms. T. Oguri for providing infectious disease consultation on the pathogenicity of the identified organisms.
Disclosure
This work was supported by JSPS KAKENHI Grant Number 15K19294 (to TK) and 20590840 (to KI) from the Japan Society for the Promotion of Science. The authors report no potential conflicts of interest relevant to this article.
1. Weinstein MP, Towns ML, Quartey SM et al. The clinical significance of positive blood cultures in the 1990s: a prospective comprehensive evaluation of the microbiology, epidemiology, and outcome of bacteremia and fungemia in adults. Clin Infect Dis. 1997;24:584-602. PubMed
2. Strand CL, Wajsbort RR, Sturmann K. Effect of iodophor vs iodine tincture skin preparation on blood culture contamination rate. JAMA. 1993;269:1004-1006. PubMed
3. Bates DW, Goldman L, Lee TH. Contaminant blood cultures and resource utilization. The true consequences of false-positive results. JAMA. 1991;265:365-369. PubMed
4. Tokuda Y, Miyasato H, Stein GH. A simple prediction algorithm for bacteraemia in patients with acute febrile illness. QJM. 2005;98:813-820. PubMed
5. Tokuda Y, Miyasato H, Stein GH, Kishaba T. The degree of chills for risk of bacteremia in acute febrile illness. Am J Med. 2005;118:1417. PubMed
6. Coburn B, Morris AM, Tomlinson G, Detsky AS. Does this adult patient with suspected bacteremia require blood cultures? JAMA. 2012;308:502-511. PubMed
7. Shapiro NI, Wolfe RE, Wright SB, Moore R, Bates DW. Who needs a blood culture? A prospectively derived and validated prediction rule. J Emerg Med. 2008;35:255-264. PubMed
8. Komatsu T, Onda T, Murayama G, et al. Predicting bacteremia based on nurse-assessed food consumption at the time of blood culture. J Hosp Med. 2012;7:702-705. PubMed
9. Henderson V. Basic Principles of Nursing Care. 2nd ed. Silver Spring, MD: American Nurses Association; 1969.
10. Therneau T, Atkinson, EJ. An Introduction to Recursive Partitioning using the RPART Routines. Mayo Foundation 2017. https://cran.r-project.org/web/packages/rpart/vignettes/longintro.pdf. Accessed May 5, 2017.
11. Pavlov VA, Wang H, Czura CJ, Friedman SG, Tracey KJ. The cholinergic anti-inflammatory pathway: a missing link in neuroimmunomodulation. Mol Med .2003;9:125-134. PubMed
12. Hansen MK, Nguyen KT, Fleshner M, et al. Effects of vagotomy on serum endotoxin, cytokines, and corticosterone after intraperitoneal lipopolysaccharide. Am J Physiol Regul Integr Comp Physiol. 2000;278:R331-336. PubMed
13. Zhang Y, Lu H, Bargmann CI. Pathogenic bacteria induce aversive olfactory learning in Caenorhabditis elegans. Nature 2005;438:179-84. PubMed
14. Van Dissel JT, Schijf V, Vogtlander N, Hoogendoorn M, van’t Wout J. Implications of chills. Lancet 1998;352:374. PubMed
15. Fukui S, Uehara Y, Fujibayashi K, et al. Bacteraemia predictive factors among general medical inpatients: a retrospective cross-sectional survey in a Japanese university hospital. BMJ Open 2016;6:e010527. PubMed
Fever in hospitalized patients is a nonspecific finding with many potential causes. Blood cultures (BC) are commonly obtained prior to commencing parenteral antibiotics in febrile patients. However, as many as 35% to 50% of positive BCs represent a contamination with organisms inoculated from the skin into culture bottles at the time of sample collection.1-3 Such results represent false-positive BCs that can lead to unnecessary investigations and treatment.
Recently, Coburn et al. reviewed the severity of chills (graded on an ordinal scale) as the most useful predictor of true bacteremia (positive likelihood ratio [LR], 4.7; 95% confidence interval [CI], 3.0–7.2),4-6 and the lack of the systemic inflammatory response syndrome (SIRS) criteria as the best negative indicator of true bacteremia with a negative LR of 0.09 (95% CI, 0.03-0.3).6,7 We have also previously reported normal food consumption as a negative indicator of true bacteremia, with a 98.3% negative predictive value.8 Henderson’s Basic Principles of Nursing Care emphasizes the importance of evaluating whether a patient can eat and drink adequately,9 and the evaluation of a patient’s food consumption is a routine nursing staff practice, which is treated as vital sign in Japan, in contrast to nursing practices in the United States.
However, these data were the result of a single-center retrospective study using the nursing staff’s assessment of food consumption, and they cannot be generalized to larger patient populations. Therefore, the aim of this prospective, multicenter study was to measure the accuracy of food consumption and shaking chills as predictive factors for true bacteremia.
METHODS
Study Design
This was a prospective multicenter observational study (UMIN ID: R000013768) involving 3 hospitals in Tokyo, Japan, that enrolled consecutive patients who had BCs obtained. This study was approved by the ethical committee at Juntendo University Nerima Hospital and each of the participating centers, and the study was conducted in accordance with the Declaration of Helsinki 1971, as revised in 1983. We evaluated 2,792 consecutive hospitalized patients (mean age, 68.9 ± 17.1 years; 55.3% men) who had BCs obtained between April 2013 and August 2014, inclusive. The indication for BC acquisition was at the discretion of the treating physician. The study protocol and the indication for BCs are described in detail elsewhere.8 We excluded patients with anorexia-inducing conditions such as gastrointestinal disease, including gastrointestinal bleeding, enterocolitis, gastric ulceration, peritonitis, appendicitis, cholangitis, pancreatitis, diverticulitis, and ischemic colitis. We also excluded patients receiving chemotherapy for malignancy. In this study, true bacteremia was defined as identical organisms isolated from 2 sets of blood cultures (a set refers to one aerobic bottle and one anaerobic bottle). Moreover, even if only one set of blood cultures was acquired, when the identified pathogen could account for the clinical presentation, we also defined this as true bacteremia. Briefly, contaminants were defined as organisms common to skin flora, including Bacillus species, coagulase-negative Staphylococcus, Corynebacterium species, and Micrococcus species, without isolation of an identical organism with the same antibiotic susceptibilities from another potentially infected site in a patient with incompatible clinical features and no risk factors for infection with the isolated organism. Single BCs that were positive for organisms that were unlikely to explain the patient’s symptoms were also considered as contaminants. Patients with contaminated BCs were excluded from the analyses.
Structure of Reliability Study Procedures
Nurses in the 3 different hospitals performed daily independent food consumption ratings during each patient’s stay. Interrater reliability assessments were conducted in the morning or afternoon, and none of the raters had access to the other nurses’ scores at any time. The study nurses performed simultaneous ratings during these assessments (one interacted with and rated the patient while the other observed and rated the same patient).
Prediction Variables of True Bacteremia
1. Food consumption. Assessment of food consumption has been previously described in detail.8 Briefly, we characterized the patients’ oral intake based on the meal taken immediately prior to the BCs. For example, if a fever developed at 2
2. Chills. As done previously, the physician evaluated the patient for a history of chills at the time of BCs and classified the patients into 1 of 4 grades4,5: “no chills,” the absence of any chills; “mild chills,” feeling cold, equivalent to needing an outer jacket; “moderate chills,” feeling very cold, equivalent to needing a thick blanket; and “shaking chills,” feeling extremely cold with rigors and generalized bodily shaking, even under a thick blanket. To distinguish between those patients who had shaking chills and those who did not, we divided the patients into 2 groups: the “shaking chills group” and the combination of none, mild, and moderate chills, referred to as the “negative shaking chills group.”
3. Other predictive variables. We considered the following additional predictive variables: age, gender, axillary body temperature (BT), heart rate (HR), systolic blood pressure (SBP), respiratory rate (RR), white blood cell count (WBC), and serum C-reactive protein level (CRP). These predictive variables were obtained immediately prior to the BCs. We defined SIRS based on standard criteria (HR >90 beats/m, RR >20/m, BT <36°C or >38°C, and a WBC <4 × 103 WBC/μL or >12 × 103 WBC/μL). Patients were subcategorized by age into 2 groups (≤69 years and >70 years). CRP levels were dichotomized as >10.0 mg/dL or ≤10.0 mg/dL. We reviewed the patients’ charts to determine whether they had received antibiotics. In the case of walk-in patients, we interviewed the patients regarding whether they had visited a clinic; if they had, they were questioned as to whether any antibiotic therapy had been prescribed.
Statistical Analysis
Continuous variables are presented as the mean with the associated standard deviation (SD). All potential variables predictive of true bacteremia are shown in Table 1. The variables were dichotomized by clinically meaningful thresholds and used as potential risk-adjusted variables. We calculated the sensitivity and specificity and positive and negative predictive value for each criterion. Multiple logistic regression analysis was used to select components that were significantly associated with true bacteremia (the level of statistical significance determined with maximum likelihood methods was set at P < .05). To visualize and quantify other aspects in the prediction of true bacteremia, a recursive partitioning analysis (RPA) was used to make a decision tree model for true bacteremia. This nonparametric regression method produces a classification tree following a series of nonsequential top-down binary splits. The tree-building process starts by considering a set of predictive variables and selects the variable that produces 2 subsets of participants with the greatest purity. Two factors are considered when splitting a node into its daughter nodes: the goodness of the split and the amount of impurity in the daughter nodes. The splitting process is repeated until further partitioning is no longer possible and the terminal nodes have been reached. Details on this method are discussed in Monte Carlo Calibration of Distributions of Partition Statistics (www.jmp.com).
Probability was considered significant at a value of P < .05. All statistical tests were 2-tailed. Statistical analyses were conducted by a physician (KI) and an independent statistician (JM) with the use of the SPSS® v.16.0 software package (SPSS Inc., Chicago, IL) and JMP® version 8.0.2 (SAS Institute, Cary, NC).
RESULTS
Patients Characteristics
Two thousand seven hundred and ninety-two patients met the inclusion criteria for our study, from which 849 were excluded (see Figure 1 for flow diagram). Among the remaining 1,943 patients, there were 317 patients with positive BCs, of which 221 patients (69.7%) were considered to have true-positive BCs and 96 (30.3%) were considered to have contaminated BCs. After excluding these 96 patients, 221 patients with true bacteremia (true bacteremic group) were compared with 1,626 nonbacteremic patients (nonbacteremic group; Figure 1). The baseline characteristics of the subjects are shown in Table 1. The mean BT was 38.4 ± 1.2°C in the true bacteremic group and 37.9 ± 1.0°C in the nonbacteremic group. The mean serum CRP level was 11.6 ± 9.6 mg/dL in the true bacteremic group and 7.3 ± 6.9 mg/dL in the nonbacteremic group. In the true bacteremic group, there were 6 afebrile patients, and 27 patients without leukocytosis. The pathogens identified from the true-positive BCs were Escherichia coli (n = 59, 26.7%), including extended-spectrum beta-lactamase producing species, Staphylococcus aureus (n = 36, 16.3%), including methicillin-resistant Staphylococcus aureus, and Klebsiella pneumoniae (n = 22, 10.0%; Supplemental Table 1).
The underlying clinical diagnoses in the true bacteremic group included urinary tract infection (UTI), pneumonia, abscess, catheter-related bloodstream infection (CRBSI), cellulitis, osteomyelitis, infective endocarditis (IE), chorioamnionitis, iatrogenic infection at hemodialysis puncture sites, bacterial meningitis, septic arthritis, and infection of unknown cause (Supplemental Table 2).
Interrater Reliability Testing of Food Consumption
Patients were evaluated during their hospital stays. The interrater reliability of the evaluation of food consumption was very high across all participating hospitals (Supplemental Table 3). To assess the reliability of the evaluations of food consumption, patients (separate from this main study) were selected randomly and evaluated independently by 2 nurses in 3 different hospitals. The kappa scores of agreement between the nurses at the 3 different hospitals were 0.83 (95% CI, 0.63-0.88), 0.90 (95% CI, 0.80-0.99), and 0.80 (95% CI, 0.67-0.99), respectively. The interrater reliability of food consumption evaluation by the nurses was very high at all participating hospitals.
Food Consumption
The low, moderate, and high food consumption groups consisted of 964 (52.1%), 306 (16.6%), and 577 (31.2%) patients, respectively (Table 1). Of these, 174 (18.0%), 33 (10.8%), and 14 (2.4%) patients, respectively, had true bacteremia. The presence of poor food consumption had a sensitivity of 93.7% (95% CI, 89.4%-97.9%), specificity of 34.6% (95% CI, 33.0%-36.2%), and a positive LR of 1.43 (95% CI, 1.37-1.50) for predicting true bacteremia. Conversely, the absence of poor food consumption (ie, normal food consumption) had a negative LR of 0.18 (95% CI, 0.17-0.19).
Chills
The no, mild, moderate, and shaking chills groups consisted of 1,514 (82.0%), 148 (8.0%), 53 (2.9%), and 132 (7.1%) patients, respectively (Table 1). Of these, 136 (9.0%), 25 (16.9%), 8 (15.1%), and 52 (39.4%) patients, respectively, had true bacteremia. The presence of shaking chills had a sensitivity of 23.5% (95% CI, 22.5%-24.6%), a specificity of 95.1% (95% CI, 90.7%-99.4%), and a positive LR of 4.78 (95% CI, 4.56–5.00) for predicting true bacteremia. Conversely, the absence of shaking chills had a negative LR of 0.80 (95% CI, 0.77-0.84).
Prediction Model for True Bacteremia
The components identified as significantly related to true bacteremia by multiple logistic regression analysis are indicated in Table 2. The significant predictors of true bacteremia were shaking chills (odds ratio [OR], 5.6; 95% CI, 3.6-8.6; P < .01), SBP <90 mmHg (OR, 3.1; 95% CI, 1.6-5.7; P < 01), CRP levels >10.0 mg/dL (OR, 2.2; 95% CI, 1.6-3.1; P < .01), BT <36°C or >38°C (OR, 1.8; 95% CI, 1.3-2.6; P < .01), WBC <4 × 103/μL or >12 × 103/μL (OR, 1.6; 95% CI, 1.2-2.3; P = .003), HR >90 bpm (OR, 1.5; 95% CI, 1.1-2.1; P = .021), and female (OR, 1.4; 95% CI, 1.0-1.9; P = .036). An RPA to create an ideal prediction model for patients with true bacteremia or nonbacteremia is shown in Figure 2. The original group consisted of 1,847 patients, including 221 patients with true bacteremia. The pretest probability of true bacteremia was 2.4% (14/577) for those with normal food consumption (Group 1) and 2.4% (13/552) for those with both normal food consumption and the absence of shaking chills (Group 2). Conversely, the pretest probability of true bacteremia was 16.3% (207/1270) for those with poor food consumption and 47.7% (51/107) for those with both poor food consumption and shaking chills. The patients with true bacteremia with normal food consumption and without shaking chills consisted of 4 cases of CRBSI and UTI, 2 cases of osteomyelitis, 1 case of IE, 1 case of chorioamnionitis, and 1 case for which the focus was unknown (Supplemental Table 4).
DISCUSSION
In this observational study, we evaluated if a simple algorithm using food consumption and shaking chills was useful for assessing whether a patient had true bacteremia. A 2-item screening checklist (nursing assessment of food consumption and shaking chills) had excellent statistical properties as a brief screening instrument for true bacteremia.
We have prospectively validated that food consumption, as assessed by nurses, is a reliable predictor of true bacteremia.8 A previous single-center retrospective study showed similar findings, but these could not be generalized across all institutions because of the limited nature of the study. In this multicenter study, we used 2 statistical methods to reduce selection bias. First, we performed a kappa analysis across the hospitals to evaluate the interrater reliability of the evaluation of food consumption. Second, we used an RPA (Figure 2), also known as a decision tree model. RPA is a step-by-step process by which a decision tree is constructed by either splitting or not splitting each node on the tree into 2 daughter nodes.10 By using this method, we successfully generated an ideal approach to predict true bacteremia using food consumption and shaking chills. After adjusting for food consumption and shaking chills, groups 1 to 2 had sequentially decreasing diagnoses of true bacteremia, varying from 221 patients to only 13 patients.
Appetite is influenced by many factors that are integrated by the brain, most importantly within the hypothalamus. Signals that impinge on the hypothalamic center include neural afferents, hormones, cytokines, and metabolites.11 These factors elicit “sickness behavior,” which includes a decrease in food-motivated behavior.12 Furthermore, exposure to pathogenic bacteria increases serotonin, which has been shown to decrease metabolism in
The strengths of this study include its relatively large sample size, multicenter design, uniformity of data collection across sites, and completeness of data collection from study participants. All of these factors allowed for a robust analysis.
However, there are several limitations of this study. First, the physicians or nurses asked the patients about the presence of shaking chills when they obtained the BCs. It may be difficult for patients, especially elderly patients, to provide this information promptly and accurately. Some patients did not call the nurse when they had shaking chills, and the chills were not witnessed by a healthcare provider. However, we used a more specific definition for shaking chills: a feeling of being extremely cold with rigors and generalized bodily shaking, even under a thick blanket. Second, this algorithm is not applicable to patients with immunosuppressed states because none of the hospitals involved in this study perform bone marrow or organ transplantation. Third, although we included patients with dementia in our cohort, we did not specifically evaluate performance of the algorithm in patients with this medical condition. It is possible that the algorithm would not perform well in this subset of patients owing to their unreliable appetite and food intake. Fourth, some medications may affect appetite, leading to reduced food consumption. Although we have not considered the details of medications in this study, we found that the pretest probability of true bacteremia was low for those patients with normal food consumption regardless of whether the medication affected their appetites or not. However, the question of whether medications truly affect patients’ appetites concurrently with bacteremia would need to be specifically addressed in a future study.
CONCLUSION
In conclusion, we have established a simple algorithm to identify patients with suspected true bacteremia who require the acquisition of blood cultures. This extremely simple model can enable physicians to make a rapid bedside estimation of the risk of true bacteremia.
Acknowledgment
The authors thank Drs. H. Honda and S. Saint, and Ms. A. Okada for their helpful discussions with regard to this study; Ms. M. Takigawa for the collection of data; and Ms. T. Oguri for providing infectious disease consultation on the pathogenicity of the identified organisms.
Disclosure
This work was supported by JSPS KAKENHI Grant Number 15K19294 (to TK) and 20590840 (to KI) from the Japan Society for the Promotion of Science. The authors report no potential conflicts of interest relevant to this article.
Fever in hospitalized patients is a nonspecific finding with many potential causes. Blood cultures (BC) are commonly obtained prior to commencing parenteral antibiotics in febrile patients. However, as many as 35% to 50% of positive BCs represent a contamination with organisms inoculated from the skin into culture bottles at the time of sample collection.1-3 Such results represent false-positive BCs that can lead to unnecessary investigations and treatment.
Recently, Coburn et al. reviewed the severity of chills (graded on an ordinal scale) as the most useful predictor of true bacteremia (positive likelihood ratio [LR], 4.7; 95% confidence interval [CI], 3.0–7.2),4-6 and the lack of the systemic inflammatory response syndrome (SIRS) criteria as the best negative indicator of true bacteremia with a negative LR of 0.09 (95% CI, 0.03-0.3).6,7 We have also previously reported normal food consumption as a negative indicator of true bacteremia, with a 98.3% negative predictive value.8 Henderson’s Basic Principles of Nursing Care emphasizes the importance of evaluating whether a patient can eat and drink adequately,9 and the evaluation of a patient’s food consumption is a routine nursing staff practice, which is treated as vital sign in Japan, in contrast to nursing practices in the United States.
However, these data were the result of a single-center retrospective study using the nursing staff’s assessment of food consumption, and they cannot be generalized to larger patient populations. Therefore, the aim of this prospective, multicenter study was to measure the accuracy of food consumption and shaking chills as predictive factors for true bacteremia.
METHODS
Study Design
This was a prospective multicenter observational study (UMIN ID: R000013768) involving 3 hospitals in Tokyo, Japan, that enrolled consecutive patients who had BCs obtained. This study was approved by the ethical committee at Juntendo University Nerima Hospital and each of the participating centers, and the study was conducted in accordance with the Declaration of Helsinki 1971, as revised in 1983. We evaluated 2,792 consecutive hospitalized patients (mean age, 68.9 ± 17.1 years; 55.3% men) who had BCs obtained between April 2013 and August 2014, inclusive. The indication for BC acquisition was at the discretion of the treating physician. The study protocol and the indication for BCs are described in detail elsewhere.8 We excluded patients with anorexia-inducing conditions such as gastrointestinal disease, including gastrointestinal bleeding, enterocolitis, gastric ulceration, peritonitis, appendicitis, cholangitis, pancreatitis, diverticulitis, and ischemic colitis. We also excluded patients receiving chemotherapy for malignancy. In this study, true bacteremia was defined as identical organisms isolated from 2 sets of blood cultures (a set refers to one aerobic bottle and one anaerobic bottle). Moreover, even if only one set of blood cultures was acquired, when the identified pathogen could account for the clinical presentation, we also defined this as true bacteremia. Briefly, contaminants were defined as organisms common to skin flora, including Bacillus species, coagulase-negative Staphylococcus, Corynebacterium species, and Micrococcus species, without isolation of an identical organism with the same antibiotic susceptibilities from another potentially infected site in a patient with incompatible clinical features and no risk factors for infection with the isolated organism. Single BCs that were positive for organisms that were unlikely to explain the patient’s symptoms were also considered as contaminants. Patients with contaminated BCs were excluded from the analyses.
Structure of Reliability Study Procedures
Nurses in the 3 different hospitals performed daily independent food consumption ratings during each patient’s stay. Interrater reliability assessments were conducted in the morning or afternoon, and none of the raters had access to the other nurses’ scores at any time. The study nurses performed simultaneous ratings during these assessments (one interacted with and rated the patient while the other observed and rated the same patient).
Prediction Variables of True Bacteremia
1. Food consumption. Assessment of food consumption has been previously described in detail.8 Briefly, we characterized the patients’ oral intake based on the meal taken immediately prior to the BCs. For example, if a fever developed at 2
2. Chills. As done previously, the physician evaluated the patient for a history of chills at the time of BCs and classified the patients into 1 of 4 grades4,5: “no chills,” the absence of any chills; “mild chills,” feeling cold, equivalent to needing an outer jacket; “moderate chills,” feeling very cold, equivalent to needing a thick blanket; and “shaking chills,” feeling extremely cold with rigors and generalized bodily shaking, even under a thick blanket. To distinguish between those patients who had shaking chills and those who did not, we divided the patients into 2 groups: the “shaking chills group” and the combination of none, mild, and moderate chills, referred to as the “negative shaking chills group.”
3. Other predictive variables. We considered the following additional predictive variables: age, gender, axillary body temperature (BT), heart rate (HR), systolic blood pressure (SBP), respiratory rate (RR), white blood cell count (WBC), and serum C-reactive protein level (CRP). These predictive variables were obtained immediately prior to the BCs. We defined SIRS based on standard criteria (HR >90 beats/m, RR >20/m, BT <36°C or >38°C, and a WBC <4 × 103 WBC/μL or >12 × 103 WBC/μL). Patients were subcategorized by age into 2 groups (≤69 years and >70 years). CRP levels were dichotomized as >10.0 mg/dL or ≤10.0 mg/dL. We reviewed the patients’ charts to determine whether they had received antibiotics. In the case of walk-in patients, we interviewed the patients regarding whether they had visited a clinic; if they had, they were questioned as to whether any antibiotic therapy had been prescribed.
Statistical Analysis
Continuous variables are presented as the mean with the associated standard deviation (SD). All potential variables predictive of true bacteremia are shown in Table 1. The variables were dichotomized by clinically meaningful thresholds and used as potential risk-adjusted variables. We calculated the sensitivity and specificity and positive and negative predictive value for each criterion. Multiple logistic regression analysis was used to select components that were significantly associated with true bacteremia (the level of statistical significance determined with maximum likelihood methods was set at P < .05). To visualize and quantify other aspects in the prediction of true bacteremia, a recursive partitioning analysis (RPA) was used to make a decision tree model for true bacteremia. This nonparametric regression method produces a classification tree following a series of nonsequential top-down binary splits. The tree-building process starts by considering a set of predictive variables and selects the variable that produces 2 subsets of participants with the greatest purity. Two factors are considered when splitting a node into its daughter nodes: the goodness of the split and the amount of impurity in the daughter nodes. The splitting process is repeated until further partitioning is no longer possible and the terminal nodes have been reached. Details on this method are discussed in Monte Carlo Calibration of Distributions of Partition Statistics (www.jmp.com).
Probability was considered significant at a value of P < .05. All statistical tests were 2-tailed. Statistical analyses were conducted by a physician (KI) and an independent statistician (JM) with the use of the SPSS® v.16.0 software package (SPSS Inc., Chicago, IL) and JMP® version 8.0.2 (SAS Institute, Cary, NC).
RESULTS
Patients Characteristics
Two thousand seven hundred and ninety-two patients met the inclusion criteria for our study, from which 849 were excluded (see Figure 1 for flow diagram). Among the remaining 1,943 patients, there were 317 patients with positive BCs, of which 221 patients (69.7%) were considered to have true-positive BCs and 96 (30.3%) were considered to have contaminated BCs. After excluding these 96 patients, 221 patients with true bacteremia (true bacteremic group) were compared with 1,626 nonbacteremic patients (nonbacteremic group; Figure 1). The baseline characteristics of the subjects are shown in Table 1. The mean BT was 38.4 ± 1.2°C in the true bacteremic group and 37.9 ± 1.0°C in the nonbacteremic group. The mean serum CRP level was 11.6 ± 9.6 mg/dL in the true bacteremic group and 7.3 ± 6.9 mg/dL in the nonbacteremic group. In the true bacteremic group, there were 6 afebrile patients, and 27 patients without leukocytosis. The pathogens identified from the true-positive BCs were Escherichia coli (n = 59, 26.7%), including extended-spectrum beta-lactamase producing species, Staphylococcus aureus (n = 36, 16.3%), including methicillin-resistant Staphylococcus aureus, and Klebsiella pneumoniae (n = 22, 10.0%; Supplemental Table 1).
The underlying clinical diagnoses in the true bacteremic group included urinary tract infection (UTI), pneumonia, abscess, catheter-related bloodstream infection (CRBSI), cellulitis, osteomyelitis, infective endocarditis (IE), chorioamnionitis, iatrogenic infection at hemodialysis puncture sites, bacterial meningitis, septic arthritis, and infection of unknown cause (Supplemental Table 2).
Interrater Reliability Testing of Food Consumption
Patients were evaluated during their hospital stays. The interrater reliability of the evaluation of food consumption was very high across all participating hospitals (Supplemental Table 3). To assess the reliability of the evaluations of food consumption, patients (separate from this main study) were selected randomly and evaluated independently by 2 nurses in 3 different hospitals. The kappa scores of agreement between the nurses at the 3 different hospitals were 0.83 (95% CI, 0.63-0.88), 0.90 (95% CI, 0.80-0.99), and 0.80 (95% CI, 0.67-0.99), respectively. The interrater reliability of food consumption evaluation by the nurses was very high at all participating hospitals.
Food Consumption
The low, moderate, and high food consumption groups consisted of 964 (52.1%), 306 (16.6%), and 577 (31.2%) patients, respectively (Table 1). Of these, 174 (18.0%), 33 (10.8%), and 14 (2.4%) patients, respectively, had true bacteremia. The presence of poor food consumption had a sensitivity of 93.7% (95% CI, 89.4%-97.9%), specificity of 34.6% (95% CI, 33.0%-36.2%), and a positive LR of 1.43 (95% CI, 1.37-1.50) for predicting true bacteremia. Conversely, the absence of poor food consumption (ie, normal food consumption) had a negative LR of 0.18 (95% CI, 0.17-0.19).
Chills
The no, mild, moderate, and shaking chills groups consisted of 1,514 (82.0%), 148 (8.0%), 53 (2.9%), and 132 (7.1%) patients, respectively (Table 1). Of these, 136 (9.0%), 25 (16.9%), 8 (15.1%), and 52 (39.4%) patients, respectively, had true bacteremia. The presence of shaking chills had a sensitivity of 23.5% (95% CI, 22.5%-24.6%), a specificity of 95.1% (95% CI, 90.7%-99.4%), and a positive LR of 4.78 (95% CI, 4.56–5.00) for predicting true bacteremia. Conversely, the absence of shaking chills had a negative LR of 0.80 (95% CI, 0.77-0.84).
Prediction Model for True Bacteremia
The components identified as significantly related to true bacteremia by multiple logistic regression analysis are indicated in Table 2. The significant predictors of true bacteremia were shaking chills (odds ratio [OR], 5.6; 95% CI, 3.6-8.6; P < .01), SBP <90 mmHg (OR, 3.1; 95% CI, 1.6-5.7; P < 01), CRP levels >10.0 mg/dL (OR, 2.2; 95% CI, 1.6-3.1; P < .01), BT <36°C or >38°C (OR, 1.8; 95% CI, 1.3-2.6; P < .01), WBC <4 × 103/μL or >12 × 103/μL (OR, 1.6; 95% CI, 1.2-2.3; P = .003), HR >90 bpm (OR, 1.5; 95% CI, 1.1-2.1; P = .021), and female (OR, 1.4; 95% CI, 1.0-1.9; P = .036). An RPA to create an ideal prediction model for patients with true bacteremia or nonbacteremia is shown in Figure 2. The original group consisted of 1,847 patients, including 221 patients with true bacteremia. The pretest probability of true bacteremia was 2.4% (14/577) for those with normal food consumption (Group 1) and 2.4% (13/552) for those with both normal food consumption and the absence of shaking chills (Group 2). Conversely, the pretest probability of true bacteremia was 16.3% (207/1270) for those with poor food consumption and 47.7% (51/107) for those with both poor food consumption and shaking chills. The patients with true bacteremia with normal food consumption and without shaking chills consisted of 4 cases of CRBSI and UTI, 2 cases of osteomyelitis, 1 case of IE, 1 case of chorioamnionitis, and 1 case for which the focus was unknown (Supplemental Table 4).
DISCUSSION
In this observational study, we evaluated if a simple algorithm using food consumption and shaking chills was useful for assessing whether a patient had true bacteremia. A 2-item screening checklist (nursing assessment of food consumption and shaking chills) had excellent statistical properties as a brief screening instrument for true bacteremia.
We have prospectively validated that food consumption, as assessed by nurses, is a reliable predictor of true bacteremia.8 A previous single-center retrospective study showed similar findings, but these could not be generalized across all institutions because of the limited nature of the study. In this multicenter study, we used 2 statistical methods to reduce selection bias. First, we performed a kappa analysis across the hospitals to evaluate the interrater reliability of the evaluation of food consumption. Second, we used an RPA (Figure 2), also known as a decision tree model. RPA is a step-by-step process by which a decision tree is constructed by either splitting or not splitting each node on the tree into 2 daughter nodes.10 By using this method, we successfully generated an ideal approach to predict true bacteremia using food consumption and shaking chills. After adjusting for food consumption and shaking chills, groups 1 to 2 had sequentially decreasing diagnoses of true bacteremia, varying from 221 patients to only 13 patients.
Appetite is influenced by many factors that are integrated by the brain, most importantly within the hypothalamus. Signals that impinge on the hypothalamic center include neural afferents, hormones, cytokines, and metabolites.11 These factors elicit “sickness behavior,” which includes a decrease in food-motivated behavior.12 Furthermore, exposure to pathogenic bacteria increases serotonin, which has been shown to decrease metabolism in
The strengths of this study include its relatively large sample size, multicenter design, uniformity of data collection across sites, and completeness of data collection from study participants. All of these factors allowed for a robust analysis.
However, there are several limitations of this study. First, the physicians or nurses asked the patients about the presence of shaking chills when they obtained the BCs. It may be difficult for patients, especially elderly patients, to provide this information promptly and accurately. Some patients did not call the nurse when they had shaking chills, and the chills were not witnessed by a healthcare provider. However, we used a more specific definition for shaking chills: a feeling of being extremely cold with rigors and generalized bodily shaking, even under a thick blanket. Second, this algorithm is not applicable to patients with immunosuppressed states because none of the hospitals involved in this study perform bone marrow or organ transplantation. Third, although we included patients with dementia in our cohort, we did not specifically evaluate performance of the algorithm in patients with this medical condition. It is possible that the algorithm would not perform well in this subset of patients owing to their unreliable appetite and food intake. Fourth, some medications may affect appetite, leading to reduced food consumption. Although we have not considered the details of medications in this study, we found that the pretest probability of true bacteremia was low for those patients with normal food consumption regardless of whether the medication affected their appetites or not. However, the question of whether medications truly affect patients’ appetites concurrently with bacteremia would need to be specifically addressed in a future study.
CONCLUSION
In conclusion, we have established a simple algorithm to identify patients with suspected true bacteremia who require the acquisition of blood cultures. This extremely simple model can enable physicians to make a rapid bedside estimation of the risk of true bacteremia.
Acknowledgment
The authors thank Drs. H. Honda and S. Saint, and Ms. A. Okada for their helpful discussions with regard to this study; Ms. M. Takigawa for the collection of data; and Ms. T. Oguri for providing infectious disease consultation on the pathogenicity of the identified organisms.
Disclosure
This work was supported by JSPS KAKENHI Grant Number 15K19294 (to TK) and 20590840 (to KI) from the Japan Society for the Promotion of Science. The authors report no potential conflicts of interest relevant to this article.
1. Weinstein MP, Towns ML, Quartey SM et al. The clinical significance of positive blood cultures in the 1990s: a prospective comprehensive evaluation of the microbiology, epidemiology, and outcome of bacteremia and fungemia in adults. Clin Infect Dis. 1997;24:584-602. PubMed
2. Strand CL, Wajsbort RR, Sturmann K. Effect of iodophor vs iodine tincture skin preparation on blood culture contamination rate. JAMA. 1993;269:1004-1006. PubMed
3. Bates DW, Goldman L, Lee TH. Contaminant blood cultures and resource utilization. The true consequences of false-positive results. JAMA. 1991;265:365-369. PubMed
4. Tokuda Y, Miyasato H, Stein GH. A simple prediction algorithm for bacteraemia in patients with acute febrile illness. QJM. 2005;98:813-820. PubMed
5. Tokuda Y, Miyasato H, Stein GH, Kishaba T. The degree of chills for risk of bacteremia in acute febrile illness. Am J Med. 2005;118:1417. PubMed
6. Coburn B, Morris AM, Tomlinson G, Detsky AS. Does this adult patient with suspected bacteremia require blood cultures? JAMA. 2012;308:502-511. PubMed
7. Shapiro NI, Wolfe RE, Wright SB, Moore R, Bates DW. Who needs a blood culture? A prospectively derived and validated prediction rule. J Emerg Med. 2008;35:255-264. PubMed
8. Komatsu T, Onda T, Murayama G, et al. Predicting bacteremia based on nurse-assessed food consumption at the time of blood culture. J Hosp Med. 2012;7:702-705. PubMed
9. Henderson V. Basic Principles of Nursing Care. 2nd ed. Silver Spring, MD: American Nurses Association; 1969.
10. Therneau T, Atkinson, EJ. An Introduction to Recursive Partitioning using the RPART Routines. Mayo Foundation 2017. https://cran.r-project.org/web/packages/rpart/vignettes/longintro.pdf. Accessed May 5, 2017.
11. Pavlov VA, Wang H, Czura CJ, Friedman SG, Tracey KJ. The cholinergic anti-inflammatory pathway: a missing link in neuroimmunomodulation. Mol Med .2003;9:125-134. PubMed
12. Hansen MK, Nguyen KT, Fleshner M, et al. Effects of vagotomy on serum endotoxin, cytokines, and corticosterone after intraperitoneal lipopolysaccharide. Am J Physiol Regul Integr Comp Physiol. 2000;278:R331-336. PubMed
13. Zhang Y, Lu H, Bargmann CI. Pathogenic bacteria induce aversive olfactory learning in Caenorhabditis elegans. Nature 2005;438:179-84. PubMed
14. Van Dissel JT, Schijf V, Vogtlander N, Hoogendoorn M, van’t Wout J. Implications of chills. Lancet 1998;352:374. PubMed
15. Fukui S, Uehara Y, Fujibayashi K, et al. Bacteraemia predictive factors among general medical inpatients: a retrospective cross-sectional survey in a Japanese university hospital. BMJ Open 2016;6:e010527. PubMed
1. Weinstein MP, Towns ML, Quartey SM et al. The clinical significance of positive blood cultures in the 1990s: a prospective comprehensive evaluation of the microbiology, epidemiology, and outcome of bacteremia and fungemia in adults. Clin Infect Dis. 1997;24:584-602. PubMed
2. Strand CL, Wajsbort RR, Sturmann K. Effect of iodophor vs iodine tincture skin preparation on blood culture contamination rate. JAMA. 1993;269:1004-1006. PubMed
3. Bates DW, Goldman L, Lee TH. Contaminant blood cultures and resource utilization. The true consequences of false-positive results. JAMA. 1991;265:365-369. PubMed
4. Tokuda Y, Miyasato H, Stein GH. A simple prediction algorithm for bacteraemia in patients with acute febrile illness. QJM. 2005;98:813-820. PubMed
5. Tokuda Y, Miyasato H, Stein GH, Kishaba T. The degree of chills for risk of bacteremia in acute febrile illness. Am J Med. 2005;118:1417. PubMed
6. Coburn B, Morris AM, Tomlinson G, Detsky AS. Does this adult patient with suspected bacteremia require blood cultures? JAMA. 2012;308:502-511. PubMed
7. Shapiro NI, Wolfe RE, Wright SB, Moore R, Bates DW. Who needs a blood culture? A prospectively derived and validated prediction rule. J Emerg Med. 2008;35:255-264. PubMed
8. Komatsu T, Onda T, Murayama G, et al. Predicting bacteremia based on nurse-assessed food consumption at the time of blood culture. J Hosp Med. 2012;7:702-705. PubMed
9. Henderson V. Basic Principles of Nursing Care. 2nd ed. Silver Spring, MD: American Nurses Association; 1969.
10. Therneau T, Atkinson, EJ. An Introduction to Recursive Partitioning using the RPART Routines. Mayo Foundation 2017. https://cran.r-project.org/web/packages/rpart/vignettes/longintro.pdf. Accessed May 5, 2017.
11. Pavlov VA, Wang H, Czura CJ, Friedman SG, Tracey KJ. The cholinergic anti-inflammatory pathway: a missing link in neuroimmunomodulation. Mol Med .2003;9:125-134. PubMed
12. Hansen MK, Nguyen KT, Fleshner M, et al. Effects of vagotomy on serum endotoxin, cytokines, and corticosterone after intraperitoneal lipopolysaccharide. Am J Physiol Regul Integr Comp Physiol. 2000;278:R331-336. PubMed
13. Zhang Y, Lu H, Bargmann CI. Pathogenic bacteria induce aversive olfactory learning in Caenorhabditis elegans. Nature 2005;438:179-84. PubMed
14. Van Dissel JT, Schijf V, Vogtlander N, Hoogendoorn M, van’t Wout J. Implications of chills. Lancet 1998;352:374. PubMed
15. Fukui S, Uehara Y, Fujibayashi K, et al. Bacteraemia predictive factors among general medical inpatients: a retrospective cross-sectional survey in a Japanese university hospital. BMJ Open 2016;6:e010527. PubMed
© 2017 Society of Hospital Medicine
Prediction Rule of Bacteremia
Fever is a nonspecific phenomenon that can result from many inciting causes such as infection, inflammation, malignancy, thromboembolic disease, drugs, and endocrine disease. In hospitalized patients, one of the most important clinical considerations is bacteremia. Although vital signs compose 3 of the 4 current criteria for the diagnosis of Systemic Inflammatory Response Syndrome (SIRS),1, 2 they contribute little to the diagnosis of the cause, which can be inflammation or infection. Unfortunately, the physician's clinical diagnosis of bacteremia lacks both sensitivity and specificity.35 Blood culture acquisition is a simple and basic diagnostic procedure routinely used in clinical practice that yields essential information for the evaluation of various infectious diseases.6 Positive blood cultures can demonstrate not only an infectious cause of disease but also the microbiological response to antibiotic therapy.7 However, studies have reported that 35% to 50% of positive blood cultures are falsely positive owing to contamination.711 False‐positive cultures may lead to the use of inappropriate or unnecessary antibiotics, additional testing and consultation, and prolonged hospitalizations that increase patient care costs.9, 12
Nursing staff caring for patients are generally able to assess oral intake, general clinical state, and care requirements. Moreover, the nursing staff are often able to identify problems with patients before physicians.13 In Japan, nurse‐assessed food consumption of every meal is standardized, and is frequently regarded as an indicator of the patient's clinical status, akin to a vital sign. In this context, we hypothesized that quantitative variations in food consumption could accurately distinguish those patients with or without bacteremia.
MATERIALS AND METHODS
Study Design
Between 2005 and 2009, we conducted a cross‐sectional observational study at Juntendo University Nerima Hospital in Tokyo, Japan. We evaluated 1179 consecutive Japanese patients (mean age, 67.8 16.8 years; 51% male) who underwent blood cultures. Patients with anorexia‐inducing conditions, such as gastrointestinal disease and those who were receiving chemotherapy for malignancy, were excluded. We also excluded patients who were not allowed to eat a regular diet. Patients aged <6 years old were also excluded. The indication for blood culture acquisition was at the discretion of the treating physicians. In general, when an axillary temperature >37.538C developed, blood cultures were taken. The study was approved by the ethics committee of Juntendo University Nerima Hospital, and was conducted in accordance with the Helsinki Declaration of 1971, as revised in 1983.
Definition of Bacteremia
In this study, bacteremia was defined as follows:
Identical organisms isolated from 2 sets of blood cultures (a set refers to 1 aerobic bottle and 1 anaerobic bottle).
If only 1 set of blood cultures was acquired and was positive for a pathogenic organism (such as enteric Gram‐negative bacilli or Streptococcus pneumonia) that could account for the clinical presentation, then the culture was considered positive.7, 14, 15
Definition of Contamination
We considered as contaminants organisms common to skin flora, including Bacillus species, coagulase‐negative staphylococci, Corynebacterium species, and Micrococcus species, without isolation of an identical organism with the same antibiotic susceptibilities from another potentially infected site in a patient with incompatible clinical features and no attributable risks.16 Single blood cultures positive for organisms thought unlikely to explain the patient's symptoms were also considered contaminants.
Assessment of Food Consumption and Inter‐Assessor Reliability
Nursing staff assessed the patients' food consumption by estimating the percentage intake at each meal, and we characterized the patients' oral intake based on the meal immediately prior to the blood culture. We categorized the patients into 3 groups: low food consumption group (<50% consumed), moderate food consumption group (>50% to <80% consumed), and high food consumption group (>80% food consumed). To assess the reliability of the evaluations of food consumption, 100 patients (separate from this main study) were selected randomly and evaluated independently by 2 nurses. The kappa score of agreement between the nurses was 0.79 (95% confidence interval [CI], 0.770.80) indicating a high level of concordance.
Other Predictor Variables
In addition to food consumption, we considered the following additional predictor variables: age, leukocyte count, C‐reactive protein (CRP), systolic blood pressure, heart rate, and body temperature.17 These predictor variables were obtained just prior to the blood culture acquisition. We defined systemic inflammatory response syndrome (SIRS) based on standard criteria (heart rate of 90 beats/min, temperature of 36 or 38C, and leukocyte level of 4000 or 12,000 cells/mL), and sepsis as SIRS in the context of clinical evidence or microbiological findings suggesting a primary focus of infection. Two investigators independently determined whether sepsis was present in each case, and the differences were resolved by consensus. Age subclassifications were categorized into 2 groups (<69 years and >70 years). CRP levels were dichotomized as above or below 10.0 mg/dL.
Statistical Analysis
Continuous variables were presented as medians with the associated interquartile range. Univariate analysis was performed using the Student's t test for continuous variables and the Pearson chi‐square test for categorical variables. Locally weighted regression analysis was applied for continuous variables significantly predictive of the outcome in univariate analysis, and the log odds of the outcome was performed to explore which cut‐off points were the best predictors of true‐positive blood culture results.18 Evaluation of best fit was performed using a multivariate logistic regression model with a forward stepwise procedure, with significant multivariate predictors of the outcome kept in the model and expressed as adjusted odds ratios. Calibration was evaluated using the Hosmer‐Lemeshow goodness‐of‐fit test. We calculated the sensitivity and specificity, and positive and negative predictive value for criteria to predict bacteremia. As a subgroup analysis, we repeated the above analytic approach after excluding those patients exposed to antibacterial drugs (which might independently impact food intake). All hypothesis testing was 2‐tailed, and P values of less than 0.05 were considered statistically significant. Statistical analysis was performed using the SPSS v.16.0 software package (SPSS Inc, Chicago, IL).
RESULTS
During the study period, 851 patients aged 16 to 99 years (66.8 16.6), were eligible for inclusion (Figure 1). Baseline characteristics of the subjects are given in Table 1. The mean body temperature ( standard deviation [SD]) was 38.1 1.1C, and the mean CRP level was 8.7 8.1 mg/dL. The results show that the patients had at least 2 SIRS criteria with elevations in temperature and heart rate. Of the 851 patients entered into the study, only 122 (14.3%) had positive blood cultures. Of these, 75 patients (8.8%) were considered to have true‐positive blood culture. In this study, blood cultures were taken at the time of onset of fever, whether that was a new inpatient admission, or during the course of an admission to the hospital. On average, blood cultures were drawn 12 days after admission (SD, 5.6 days). Despite the variation in onset of fever, the inverse relationship of blood culture positivity to decreased food consumption held true (data not shown). Gram‐positive and Gram‐negative organisms were obtained in near equal amounts. The main pathogens recovered from the true‐positive blood cultures were Gram‐positive cocci (26 patients [34.7% in true‐positive blood cultures]), and Gram‐negative bacilli (46 patients [61.3%]), as shown in Table 1. The underlying clinical diagnosis included 28 urinary tract infections; 9 catheter‐associated infections; 5 cases each of pneumonia and abscess; 3 cases of phlebitis; 2 cases of meningitis and osteomyelitis; 1 case each of infective endocarditis, decubitus ulcer, and pelvic infection; and 17 cases of infection with an unknown focus.
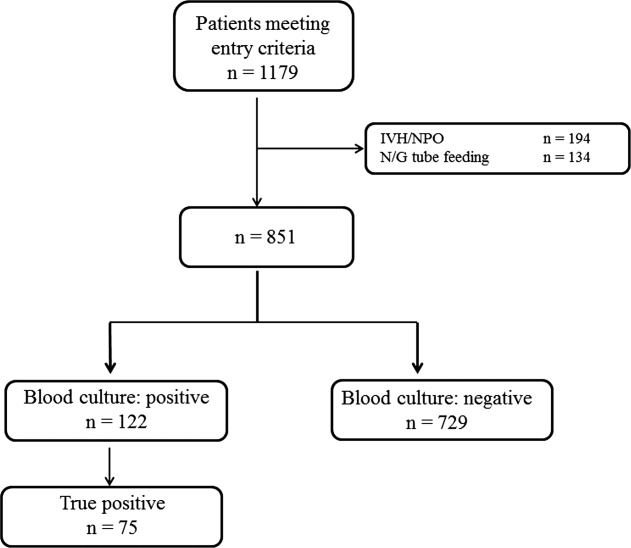
Mean | SD | |
---|---|---|
| ||
Age, years | 66.8 | 16.7 |
Male (%) | 50.6 | |
Vital signs | ||
Systolic blood pressure, mmHg | 122.6 | 25.9 |
Diastolic blood pressure, mmHg | 65.3 | 14.6 |
Heart rate, beats/min | 91.1 | 19.2 |
Body temperature, C | 38.1 | 1.1 |
Laboratory results | ||
Leukocyte, 100 /L | 10.6 | 11.8 |
C‐reactive protein, mg/L | 8.8 | 8.1 |
Results of blood cultures | N | % |
Blood culture positive | 122 | 14.3 |
True positive | 75 | 8.8 |
Gram‐positive coccus | 26 | 3.1 |
Gram‐negative baccili | 46 | 5.4 |
Gram‐negative coccus | 1 | 0.1 |
Fungus | 1 | 0.1 |
Anaerobic | 1 | 0.1 |
Contamination | 47 | 5.6 |
Blood culture negative | 729 | 85.7 |
Food consumption | ||
Low food consumption group | 344 | 4.4 |
Moderate food consumption group | 152 | 17.9 |
High food consumption group | 354 | 41.4 |
Low, moderate, and high food consumption groups consisted of 344 patients (40.4%), 152 patients (17.9%), and 354 patients (41.7%), respectively (Table 1). Of these, 63 patients, 6 patients, and 6 patients had bacteremia in the low, moderate, and high food consumption group, respectively. In order to distinguish those patients who had decreased food consumption compared to almost normal food consumption, low and moderate food consumption groups were combined and compared to the high food consumption group. Comparison of the combined low and moderate food consumption group versus the high food consumption group revealed a sensitivity of 92.0% and a negative predictive value of 98.3% for excluding true bacteremia. Conversely, the specificity (45.1%) and the positive predictive value (13.9%) were poor.
In the univariate analysis, the following variables were not statistically significantly associated with true bacteremia: age, heart rate, and leukocyte counts. Significant univariate predictors of bacteremia and their associated cut‐off points were body temperature of 36 or 38C (odds ratio [OR], 2.5; 95% CI, 1.54.4), CRP 10.0 mg/dL (OR, 2.0; 95% CI, 1.23.2), and food consumption (OR, 8.5; 95% CI, 3.818.6) (Table 2). There was no evidence of colinearity. In the final stepwise logistic regression (Table 3), the significant predictors of bacteremia were body temperature of 36 or 38C (OR, 2.4; 95% CI, 1.44.2; P = 0.002), C‐reactive protein of 10.0 mg/dL (OR, 1.9; 95% CI, 1.23.0; P = 0.011), and food consumption (OR, 7.5; 95% CI, 3.416.6; P < 0.001). We identified only 6 patients with bacteremia in the high food consumption group. Three of the patients had been previously treated with antibiotics for conditions including infective endocarditis, osteomyelitis, and myelodysplasic syndrome.
Variables | Blood Culture Result | P Value | OR (95% CI) | |
---|---|---|---|---|
Negative (n = 729) (%) | Positive (n = 75) | |||
| ||||
Age, years | 66.6 | 69.0 | ||
Mean SD | 16.9 | 13.5 | ||
70 | 408 (56.0) | 43 (57.3) | 0.7 | |
Heart rate, beats/min | 90.5 | 96.3 | ||
Mean SD | 19.0 | 20.3 | ||
90 | 368 (50.4) | 43 (57.3) | 0.3 | |
Temperature, C | 38.0 | 38.6 | ||
Mean SD | 1.0 | 1.6 | ||
36, 38 | 444 (61.0) | 61 (81.3) | <0.001 | 2.5 (1.54.4) |
Leukocyte count, cells/L | 10.1 | 11.2 | ||
Mean SD, 100 | 12.1 | 7.4 | ||
120 103, <4 103 | 336 (46.1) | 38 (50.7) | 0.4 | |
C‐reactive protein | ||||
Mean SD | 7.8 | 10.0 | ||
10.0 | 245 (33.6) | 39 (52.0) | 0.0004 | 2.0 (1.23.2) |
Food consumption | ||||
Low and moderate | 426 (58.9) | 69 (92.0) | ||
High | 350 (48.0) | 6 (8.0) | <0.001 | 8.5 (3.818.6) |
Variables | OR (95% CI) | P Value |
---|---|---|
| ||
Temperature, C 36 or 38 | 2.4 (1.44.2) | 0.002 |
C‐reactive protein, mg/dL 10.0 | 1.9 (1.23.0) | 0.011 |
Food consumption High vs low and moderate | 7.5 (3.416.6) | <0.001 |
On further analysis, we excluded patients who had received antibiotics before blood culture acquisition. There were 661 patients in this subanalysis. Low, moderate, and high food consumption groups consisted of 282 patients (41.4%), 118 patients (17.3%), and 261 patients (38.3%), respectively. Of these, 50 patients (17.7%), 5 patients (4.2%), and 4 patients (1.5%) had bacteremia in the low, moderate, and high food consumption groups, respectively. The sensitivity and negative predictive values were 93.2% and 98.5%, respectively. In the stepwise logistic regression, significant predictors of bacteremia were body temperature of 36 or 38C (OR, 3.0; 95% CI, 1.55.6; P = 0.001), CRP 10.0 mg/dL (OR, 2.1; 95% CI, 1.23.7; P = 0.006), and food consumption (OR, 9.3; 95% CI, 3.326.1; P < 0.001).
DISCUSSION
We found that in a group of 851 Japanese patients who were suspected with bacterial infection, the estimated food consumption was negatively associated, both significantly and independently, with the subsequent isolation of microorganisms from their blood cultures. If validated in other studies, this simple rule of thumb can provide the clinician with reasonable confidence that a febrile patient has a low probability of being bacteremic, as long as the appetite remains normal. Both the sensitivity and the negative predictive value were extremely high at 92.3% and 98.3%, respectively, suggesting that adequate oral intake is a strong marker against the presence of bacteremia. In this study, it was the strongest predictor of bacteremia in multivariate analysis. After including only antibiotic‐naive patients, the sensitivity and the negative predictive values were 93.2% and 98.5%, respectively. Administration of antibiotics may lead to improved appetite in febrile patients despite bacteremia in the presence of fever, and therefore, inquiring about recent or current antimicrobial usage should be a requirement when considering oral intake as an indicator of bacteremia.
Our study has limitations. Since we did not make treatment decisions based on oral intake, we cannot conclude that it is safe to withhold antibiotic treatment on the basis of food intake alone. Additionally, this study would need to be repeated across many different age groups and racial groups to ensure applicability to the general population. It is also unknown whether this rule would be applicable to patients with underlying immunosuppression. Finally, although inter‐rater reliability was high in our center, nurses in other settings may not be as diligent in their assessment of food consumption. The high inter‐assessor reliability in our setting, however, suggests that objective assessment of food intake can be performed reliably in settings in which accurate documentation of food consumption is expected.
In summary, we found that normal food intake was strongly and negatively associated with bacteremia in febrile patients. This observation, if validated in other settings, may serve as a simple aid to assist in the clinical diagnosis of bacteremia or for recruitment of patients with a high likelihood of bacteremia into clinical trials.
Acknowledgements
The authors thank Drs T. Morimoto and S. Ueda for assistance with statistical analysis, Ms M. Takigawa, and M. Kudo for collection of data, and Drs T. Oguri and Tachibana for infectious disease consultation on the pathogenicity of the microbiological organisms.
- 2001 SCCM/ESICM/ACCP/ATS/SIS International Sepsis Definitions Conference. Crit Care Med. 2003;31(4):1250–1256. , , , et al.
- Septic shock. Lancet. 2005;365(9453):63–78. , , .
- A simple index to identify occult bacterial infection in adults with acute unexplained fever. Arch Intern Med. 1987;147(4):666–671. , , , .
- Occult bacterial infection in adults with unexplained fever. Validation of a diagnostic index. Arch Intern Med. 1990;150(6):1270–1272. , , .
- Bacteremia in febrile patients. A clinical model for diagnosis. Arch Intern Med. 1991;151(9):1801–1806. , , , , .
- Blood cultures. Ann Intern Med. 1987;106(2):246–253. , .
- The clinical significance of positive blood cultures in the 1990s: a prospective comprehensive evaluation of the microbiology, epidemiology, and outcome of bacteremia and fungemia in adults. Clin Infect Dis. 1997;24(4):584–602. , , , et al.
- Effect of iodophor vs iodine tincture skin preparation on blood culture contamination rate. JAMA. 1993;269(8):1004–1006. , , .
- Predicting bacteremia in patients with sepsis syndrome. Academic Medical Center Consortium Sepsis Project Working Group. J Infect Dis. 1997;176(6):1538–1551. , , , et al.
- Clinical issues of blood cultures. Arch Intern Med. 1994;154(8):841–849. , .
- High frequency of pseudobacteremia at a university hospital. Infect Control Hosp Epidemiol. 1997;18(3):200–202. , , .
- Predicting bacteremia in hospitalized patients. A prospectively validated model. Ann Intern Med. 1990;113(7):495–500. , , , .
- Decisions made by critical care nurses during mechanical ventilation and weaning in an Australian intensive care unit. Am J Crit Care. 2007;16(5):434–443; quiz 444. , , , .
- Updated review of blood culture contamination. Clin Microbiol Rev. 2006;19(4):788–802. , .
- Minimizing the workup of blood culture contaminants: implementation and evaluation of a laboratory‐based algorithm. J Clin Microbiol. 2002;40(7):2437–2444. , , , et al.
- Evaluation of positive blood cultures. Guidelines for early differentiation of contaminated from valid positive cultures. Arch Intern Med. 1972;130(1):84–87. , .
- Predicting bacteremia at the bedside. Clin Infect Dis. 2004;38(3):357–362. , , , et al.
- Local Regression and Likelihood. New York, NY: Springer; 1999. .
Fever is a nonspecific phenomenon that can result from many inciting causes such as infection, inflammation, malignancy, thromboembolic disease, drugs, and endocrine disease. In hospitalized patients, one of the most important clinical considerations is bacteremia. Although vital signs compose 3 of the 4 current criteria for the diagnosis of Systemic Inflammatory Response Syndrome (SIRS),1, 2 they contribute little to the diagnosis of the cause, which can be inflammation or infection. Unfortunately, the physician's clinical diagnosis of bacteremia lacks both sensitivity and specificity.35 Blood culture acquisition is a simple and basic diagnostic procedure routinely used in clinical practice that yields essential information for the evaluation of various infectious diseases.6 Positive blood cultures can demonstrate not only an infectious cause of disease but also the microbiological response to antibiotic therapy.7 However, studies have reported that 35% to 50% of positive blood cultures are falsely positive owing to contamination.711 False‐positive cultures may lead to the use of inappropriate or unnecessary antibiotics, additional testing and consultation, and prolonged hospitalizations that increase patient care costs.9, 12
Nursing staff caring for patients are generally able to assess oral intake, general clinical state, and care requirements. Moreover, the nursing staff are often able to identify problems with patients before physicians.13 In Japan, nurse‐assessed food consumption of every meal is standardized, and is frequently regarded as an indicator of the patient's clinical status, akin to a vital sign. In this context, we hypothesized that quantitative variations in food consumption could accurately distinguish those patients with or without bacteremia.
MATERIALS AND METHODS
Study Design
Between 2005 and 2009, we conducted a cross‐sectional observational study at Juntendo University Nerima Hospital in Tokyo, Japan. We evaluated 1179 consecutive Japanese patients (mean age, 67.8 16.8 years; 51% male) who underwent blood cultures. Patients with anorexia‐inducing conditions, such as gastrointestinal disease and those who were receiving chemotherapy for malignancy, were excluded. We also excluded patients who were not allowed to eat a regular diet. Patients aged <6 years old were also excluded. The indication for blood culture acquisition was at the discretion of the treating physicians. In general, when an axillary temperature >37.538C developed, blood cultures were taken. The study was approved by the ethics committee of Juntendo University Nerima Hospital, and was conducted in accordance with the Helsinki Declaration of 1971, as revised in 1983.
Definition of Bacteremia
In this study, bacteremia was defined as follows:
Identical organisms isolated from 2 sets of blood cultures (a set refers to 1 aerobic bottle and 1 anaerobic bottle).
If only 1 set of blood cultures was acquired and was positive for a pathogenic organism (such as enteric Gram‐negative bacilli or Streptococcus pneumonia) that could account for the clinical presentation, then the culture was considered positive.7, 14, 15
Definition of Contamination
We considered as contaminants organisms common to skin flora, including Bacillus species, coagulase‐negative staphylococci, Corynebacterium species, and Micrococcus species, without isolation of an identical organism with the same antibiotic susceptibilities from another potentially infected site in a patient with incompatible clinical features and no attributable risks.16 Single blood cultures positive for organisms thought unlikely to explain the patient's symptoms were also considered contaminants.
Assessment of Food Consumption and Inter‐Assessor Reliability
Nursing staff assessed the patients' food consumption by estimating the percentage intake at each meal, and we characterized the patients' oral intake based on the meal immediately prior to the blood culture. We categorized the patients into 3 groups: low food consumption group (<50% consumed), moderate food consumption group (>50% to <80% consumed), and high food consumption group (>80% food consumed). To assess the reliability of the evaluations of food consumption, 100 patients (separate from this main study) were selected randomly and evaluated independently by 2 nurses. The kappa score of agreement between the nurses was 0.79 (95% confidence interval [CI], 0.770.80) indicating a high level of concordance.
Other Predictor Variables
In addition to food consumption, we considered the following additional predictor variables: age, leukocyte count, C‐reactive protein (CRP), systolic blood pressure, heart rate, and body temperature.17 These predictor variables were obtained just prior to the blood culture acquisition. We defined systemic inflammatory response syndrome (SIRS) based on standard criteria (heart rate of 90 beats/min, temperature of 36 or 38C, and leukocyte level of 4000 or 12,000 cells/mL), and sepsis as SIRS in the context of clinical evidence or microbiological findings suggesting a primary focus of infection. Two investigators independently determined whether sepsis was present in each case, and the differences were resolved by consensus. Age subclassifications were categorized into 2 groups (<69 years and >70 years). CRP levels were dichotomized as above or below 10.0 mg/dL.
Statistical Analysis
Continuous variables were presented as medians with the associated interquartile range. Univariate analysis was performed using the Student's t test for continuous variables and the Pearson chi‐square test for categorical variables. Locally weighted regression analysis was applied for continuous variables significantly predictive of the outcome in univariate analysis, and the log odds of the outcome was performed to explore which cut‐off points were the best predictors of true‐positive blood culture results.18 Evaluation of best fit was performed using a multivariate logistic regression model with a forward stepwise procedure, with significant multivariate predictors of the outcome kept in the model and expressed as adjusted odds ratios. Calibration was evaluated using the Hosmer‐Lemeshow goodness‐of‐fit test. We calculated the sensitivity and specificity, and positive and negative predictive value for criteria to predict bacteremia. As a subgroup analysis, we repeated the above analytic approach after excluding those patients exposed to antibacterial drugs (which might independently impact food intake). All hypothesis testing was 2‐tailed, and P values of less than 0.05 were considered statistically significant. Statistical analysis was performed using the SPSS v.16.0 software package (SPSS Inc, Chicago, IL).
RESULTS
During the study period, 851 patients aged 16 to 99 years (66.8 16.6), were eligible for inclusion (Figure 1). Baseline characteristics of the subjects are given in Table 1. The mean body temperature ( standard deviation [SD]) was 38.1 1.1C, and the mean CRP level was 8.7 8.1 mg/dL. The results show that the patients had at least 2 SIRS criteria with elevations in temperature and heart rate. Of the 851 patients entered into the study, only 122 (14.3%) had positive blood cultures. Of these, 75 patients (8.8%) were considered to have true‐positive blood culture. In this study, blood cultures were taken at the time of onset of fever, whether that was a new inpatient admission, or during the course of an admission to the hospital. On average, blood cultures were drawn 12 days after admission (SD, 5.6 days). Despite the variation in onset of fever, the inverse relationship of blood culture positivity to decreased food consumption held true (data not shown). Gram‐positive and Gram‐negative organisms were obtained in near equal amounts. The main pathogens recovered from the true‐positive blood cultures were Gram‐positive cocci (26 patients [34.7% in true‐positive blood cultures]), and Gram‐negative bacilli (46 patients [61.3%]), as shown in Table 1. The underlying clinical diagnosis included 28 urinary tract infections; 9 catheter‐associated infections; 5 cases each of pneumonia and abscess; 3 cases of phlebitis; 2 cases of meningitis and osteomyelitis; 1 case each of infective endocarditis, decubitus ulcer, and pelvic infection; and 17 cases of infection with an unknown focus.
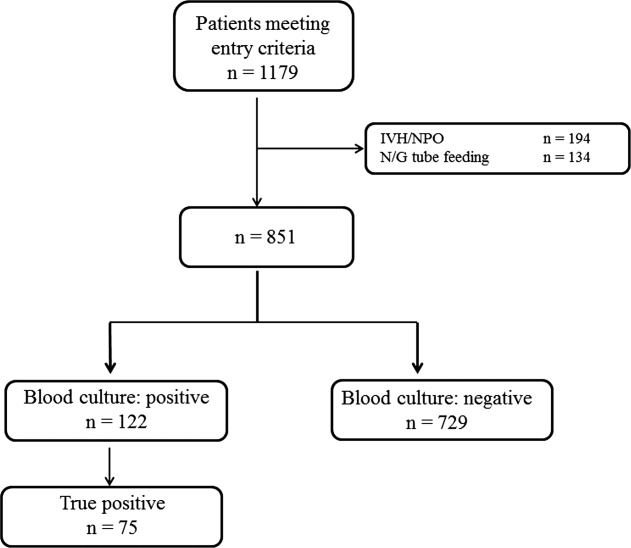
Mean | SD | |
---|---|---|
| ||
Age, years | 66.8 | 16.7 |
Male (%) | 50.6 | |
Vital signs | ||
Systolic blood pressure, mmHg | 122.6 | 25.9 |
Diastolic blood pressure, mmHg | 65.3 | 14.6 |
Heart rate, beats/min | 91.1 | 19.2 |
Body temperature, C | 38.1 | 1.1 |
Laboratory results | ||
Leukocyte, 100 /L | 10.6 | 11.8 |
C‐reactive protein, mg/L | 8.8 | 8.1 |
Results of blood cultures | N | % |
Blood culture positive | 122 | 14.3 |
True positive | 75 | 8.8 |
Gram‐positive coccus | 26 | 3.1 |
Gram‐negative baccili | 46 | 5.4 |
Gram‐negative coccus | 1 | 0.1 |
Fungus | 1 | 0.1 |
Anaerobic | 1 | 0.1 |
Contamination | 47 | 5.6 |
Blood culture negative | 729 | 85.7 |
Food consumption | ||
Low food consumption group | 344 | 4.4 |
Moderate food consumption group | 152 | 17.9 |
High food consumption group | 354 | 41.4 |
Low, moderate, and high food consumption groups consisted of 344 patients (40.4%), 152 patients (17.9%), and 354 patients (41.7%), respectively (Table 1). Of these, 63 patients, 6 patients, and 6 patients had bacteremia in the low, moderate, and high food consumption group, respectively. In order to distinguish those patients who had decreased food consumption compared to almost normal food consumption, low and moderate food consumption groups were combined and compared to the high food consumption group. Comparison of the combined low and moderate food consumption group versus the high food consumption group revealed a sensitivity of 92.0% and a negative predictive value of 98.3% for excluding true bacteremia. Conversely, the specificity (45.1%) and the positive predictive value (13.9%) were poor.
In the univariate analysis, the following variables were not statistically significantly associated with true bacteremia: age, heart rate, and leukocyte counts. Significant univariate predictors of bacteremia and their associated cut‐off points were body temperature of 36 or 38C (odds ratio [OR], 2.5; 95% CI, 1.54.4), CRP 10.0 mg/dL (OR, 2.0; 95% CI, 1.23.2), and food consumption (OR, 8.5; 95% CI, 3.818.6) (Table 2). There was no evidence of colinearity. In the final stepwise logistic regression (Table 3), the significant predictors of bacteremia were body temperature of 36 or 38C (OR, 2.4; 95% CI, 1.44.2; P = 0.002), C‐reactive protein of 10.0 mg/dL (OR, 1.9; 95% CI, 1.23.0; P = 0.011), and food consumption (OR, 7.5; 95% CI, 3.416.6; P < 0.001). We identified only 6 patients with bacteremia in the high food consumption group. Three of the patients had been previously treated with antibiotics for conditions including infective endocarditis, osteomyelitis, and myelodysplasic syndrome.
Variables | Blood Culture Result | P Value | OR (95% CI) | |
---|---|---|---|---|
Negative (n = 729) (%) | Positive (n = 75) | |||
| ||||
Age, years | 66.6 | 69.0 | ||
Mean SD | 16.9 | 13.5 | ||
70 | 408 (56.0) | 43 (57.3) | 0.7 | |
Heart rate, beats/min | 90.5 | 96.3 | ||
Mean SD | 19.0 | 20.3 | ||
90 | 368 (50.4) | 43 (57.3) | 0.3 | |
Temperature, C | 38.0 | 38.6 | ||
Mean SD | 1.0 | 1.6 | ||
36, 38 | 444 (61.0) | 61 (81.3) | <0.001 | 2.5 (1.54.4) |
Leukocyte count, cells/L | 10.1 | 11.2 | ||
Mean SD, 100 | 12.1 | 7.4 | ||
120 103, <4 103 | 336 (46.1) | 38 (50.7) | 0.4 | |
C‐reactive protein | ||||
Mean SD | 7.8 | 10.0 | ||
10.0 | 245 (33.6) | 39 (52.0) | 0.0004 | 2.0 (1.23.2) |
Food consumption | ||||
Low and moderate | 426 (58.9) | 69 (92.0) | ||
High | 350 (48.0) | 6 (8.0) | <0.001 | 8.5 (3.818.6) |
Variables | OR (95% CI) | P Value |
---|---|---|
| ||
Temperature, C 36 or 38 | 2.4 (1.44.2) | 0.002 |
C‐reactive protein, mg/dL 10.0 | 1.9 (1.23.0) | 0.011 |
Food consumption High vs low and moderate | 7.5 (3.416.6) | <0.001 |
On further analysis, we excluded patients who had received antibiotics before blood culture acquisition. There were 661 patients in this subanalysis. Low, moderate, and high food consumption groups consisted of 282 patients (41.4%), 118 patients (17.3%), and 261 patients (38.3%), respectively. Of these, 50 patients (17.7%), 5 patients (4.2%), and 4 patients (1.5%) had bacteremia in the low, moderate, and high food consumption groups, respectively. The sensitivity and negative predictive values were 93.2% and 98.5%, respectively. In the stepwise logistic regression, significant predictors of bacteremia were body temperature of 36 or 38C (OR, 3.0; 95% CI, 1.55.6; P = 0.001), CRP 10.0 mg/dL (OR, 2.1; 95% CI, 1.23.7; P = 0.006), and food consumption (OR, 9.3; 95% CI, 3.326.1; P < 0.001).
DISCUSSION
We found that in a group of 851 Japanese patients who were suspected with bacterial infection, the estimated food consumption was negatively associated, both significantly and independently, with the subsequent isolation of microorganisms from their blood cultures. If validated in other studies, this simple rule of thumb can provide the clinician with reasonable confidence that a febrile patient has a low probability of being bacteremic, as long as the appetite remains normal. Both the sensitivity and the negative predictive value were extremely high at 92.3% and 98.3%, respectively, suggesting that adequate oral intake is a strong marker against the presence of bacteremia. In this study, it was the strongest predictor of bacteremia in multivariate analysis. After including only antibiotic‐naive patients, the sensitivity and the negative predictive values were 93.2% and 98.5%, respectively. Administration of antibiotics may lead to improved appetite in febrile patients despite bacteremia in the presence of fever, and therefore, inquiring about recent or current antimicrobial usage should be a requirement when considering oral intake as an indicator of bacteremia.
Our study has limitations. Since we did not make treatment decisions based on oral intake, we cannot conclude that it is safe to withhold antibiotic treatment on the basis of food intake alone. Additionally, this study would need to be repeated across many different age groups and racial groups to ensure applicability to the general population. It is also unknown whether this rule would be applicable to patients with underlying immunosuppression. Finally, although inter‐rater reliability was high in our center, nurses in other settings may not be as diligent in their assessment of food consumption. The high inter‐assessor reliability in our setting, however, suggests that objective assessment of food intake can be performed reliably in settings in which accurate documentation of food consumption is expected.
In summary, we found that normal food intake was strongly and negatively associated with bacteremia in febrile patients. This observation, if validated in other settings, may serve as a simple aid to assist in the clinical diagnosis of bacteremia or for recruitment of patients with a high likelihood of bacteremia into clinical trials.
Acknowledgements
The authors thank Drs T. Morimoto and S. Ueda for assistance with statistical analysis, Ms M. Takigawa, and M. Kudo for collection of data, and Drs T. Oguri and Tachibana for infectious disease consultation on the pathogenicity of the microbiological organisms.
Fever is a nonspecific phenomenon that can result from many inciting causes such as infection, inflammation, malignancy, thromboembolic disease, drugs, and endocrine disease. In hospitalized patients, one of the most important clinical considerations is bacteremia. Although vital signs compose 3 of the 4 current criteria for the diagnosis of Systemic Inflammatory Response Syndrome (SIRS),1, 2 they contribute little to the diagnosis of the cause, which can be inflammation or infection. Unfortunately, the physician's clinical diagnosis of bacteremia lacks both sensitivity and specificity.35 Blood culture acquisition is a simple and basic diagnostic procedure routinely used in clinical practice that yields essential information for the evaluation of various infectious diseases.6 Positive blood cultures can demonstrate not only an infectious cause of disease but also the microbiological response to antibiotic therapy.7 However, studies have reported that 35% to 50% of positive blood cultures are falsely positive owing to contamination.711 False‐positive cultures may lead to the use of inappropriate or unnecessary antibiotics, additional testing and consultation, and prolonged hospitalizations that increase patient care costs.9, 12
Nursing staff caring for patients are generally able to assess oral intake, general clinical state, and care requirements. Moreover, the nursing staff are often able to identify problems with patients before physicians.13 In Japan, nurse‐assessed food consumption of every meal is standardized, and is frequently regarded as an indicator of the patient's clinical status, akin to a vital sign. In this context, we hypothesized that quantitative variations in food consumption could accurately distinguish those patients with or without bacteremia.
MATERIALS AND METHODS
Study Design
Between 2005 and 2009, we conducted a cross‐sectional observational study at Juntendo University Nerima Hospital in Tokyo, Japan. We evaluated 1179 consecutive Japanese patients (mean age, 67.8 16.8 years; 51% male) who underwent blood cultures. Patients with anorexia‐inducing conditions, such as gastrointestinal disease and those who were receiving chemotherapy for malignancy, were excluded. We also excluded patients who were not allowed to eat a regular diet. Patients aged <6 years old were also excluded. The indication for blood culture acquisition was at the discretion of the treating physicians. In general, when an axillary temperature >37.538C developed, blood cultures were taken. The study was approved by the ethics committee of Juntendo University Nerima Hospital, and was conducted in accordance with the Helsinki Declaration of 1971, as revised in 1983.
Definition of Bacteremia
In this study, bacteremia was defined as follows:
Identical organisms isolated from 2 sets of blood cultures (a set refers to 1 aerobic bottle and 1 anaerobic bottle).
If only 1 set of blood cultures was acquired and was positive for a pathogenic organism (such as enteric Gram‐negative bacilli or Streptococcus pneumonia) that could account for the clinical presentation, then the culture was considered positive.7, 14, 15
Definition of Contamination
We considered as contaminants organisms common to skin flora, including Bacillus species, coagulase‐negative staphylococci, Corynebacterium species, and Micrococcus species, without isolation of an identical organism with the same antibiotic susceptibilities from another potentially infected site in a patient with incompatible clinical features and no attributable risks.16 Single blood cultures positive for organisms thought unlikely to explain the patient's symptoms were also considered contaminants.
Assessment of Food Consumption and Inter‐Assessor Reliability
Nursing staff assessed the patients' food consumption by estimating the percentage intake at each meal, and we characterized the patients' oral intake based on the meal immediately prior to the blood culture. We categorized the patients into 3 groups: low food consumption group (<50% consumed), moderate food consumption group (>50% to <80% consumed), and high food consumption group (>80% food consumed). To assess the reliability of the evaluations of food consumption, 100 patients (separate from this main study) were selected randomly and evaluated independently by 2 nurses. The kappa score of agreement between the nurses was 0.79 (95% confidence interval [CI], 0.770.80) indicating a high level of concordance.
Other Predictor Variables
In addition to food consumption, we considered the following additional predictor variables: age, leukocyte count, C‐reactive protein (CRP), systolic blood pressure, heart rate, and body temperature.17 These predictor variables were obtained just prior to the blood culture acquisition. We defined systemic inflammatory response syndrome (SIRS) based on standard criteria (heart rate of 90 beats/min, temperature of 36 or 38C, and leukocyte level of 4000 or 12,000 cells/mL), and sepsis as SIRS in the context of clinical evidence or microbiological findings suggesting a primary focus of infection. Two investigators independently determined whether sepsis was present in each case, and the differences were resolved by consensus. Age subclassifications were categorized into 2 groups (<69 years and >70 years). CRP levels were dichotomized as above or below 10.0 mg/dL.
Statistical Analysis
Continuous variables were presented as medians with the associated interquartile range. Univariate analysis was performed using the Student's t test for continuous variables and the Pearson chi‐square test for categorical variables. Locally weighted regression analysis was applied for continuous variables significantly predictive of the outcome in univariate analysis, and the log odds of the outcome was performed to explore which cut‐off points were the best predictors of true‐positive blood culture results.18 Evaluation of best fit was performed using a multivariate logistic regression model with a forward stepwise procedure, with significant multivariate predictors of the outcome kept in the model and expressed as adjusted odds ratios. Calibration was evaluated using the Hosmer‐Lemeshow goodness‐of‐fit test. We calculated the sensitivity and specificity, and positive and negative predictive value for criteria to predict bacteremia. As a subgroup analysis, we repeated the above analytic approach after excluding those patients exposed to antibacterial drugs (which might independently impact food intake). All hypothesis testing was 2‐tailed, and P values of less than 0.05 were considered statistically significant. Statistical analysis was performed using the SPSS v.16.0 software package (SPSS Inc, Chicago, IL).
RESULTS
During the study period, 851 patients aged 16 to 99 years (66.8 16.6), were eligible for inclusion (Figure 1). Baseline characteristics of the subjects are given in Table 1. The mean body temperature ( standard deviation [SD]) was 38.1 1.1C, and the mean CRP level was 8.7 8.1 mg/dL. The results show that the patients had at least 2 SIRS criteria with elevations in temperature and heart rate. Of the 851 patients entered into the study, only 122 (14.3%) had positive blood cultures. Of these, 75 patients (8.8%) were considered to have true‐positive blood culture. In this study, blood cultures were taken at the time of onset of fever, whether that was a new inpatient admission, or during the course of an admission to the hospital. On average, blood cultures were drawn 12 days after admission (SD, 5.6 days). Despite the variation in onset of fever, the inverse relationship of blood culture positivity to decreased food consumption held true (data not shown). Gram‐positive and Gram‐negative organisms were obtained in near equal amounts. The main pathogens recovered from the true‐positive blood cultures were Gram‐positive cocci (26 patients [34.7% in true‐positive blood cultures]), and Gram‐negative bacilli (46 patients [61.3%]), as shown in Table 1. The underlying clinical diagnosis included 28 urinary tract infections; 9 catheter‐associated infections; 5 cases each of pneumonia and abscess; 3 cases of phlebitis; 2 cases of meningitis and osteomyelitis; 1 case each of infective endocarditis, decubitus ulcer, and pelvic infection; and 17 cases of infection with an unknown focus.
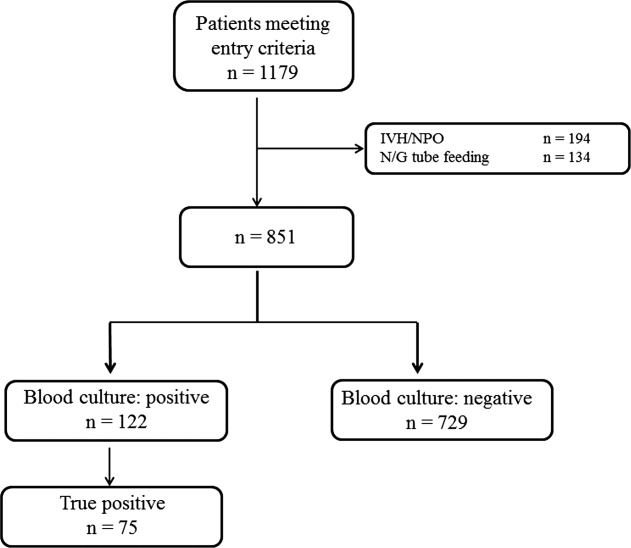
Mean | SD | |
---|---|---|
| ||
Age, years | 66.8 | 16.7 |
Male (%) | 50.6 | |
Vital signs | ||
Systolic blood pressure, mmHg | 122.6 | 25.9 |
Diastolic blood pressure, mmHg | 65.3 | 14.6 |
Heart rate, beats/min | 91.1 | 19.2 |
Body temperature, C | 38.1 | 1.1 |
Laboratory results | ||
Leukocyte, 100 /L | 10.6 | 11.8 |
C‐reactive protein, mg/L | 8.8 | 8.1 |
Results of blood cultures | N | % |
Blood culture positive | 122 | 14.3 |
True positive | 75 | 8.8 |
Gram‐positive coccus | 26 | 3.1 |
Gram‐negative baccili | 46 | 5.4 |
Gram‐negative coccus | 1 | 0.1 |
Fungus | 1 | 0.1 |
Anaerobic | 1 | 0.1 |
Contamination | 47 | 5.6 |
Blood culture negative | 729 | 85.7 |
Food consumption | ||
Low food consumption group | 344 | 4.4 |
Moderate food consumption group | 152 | 17.9 |
High food consumption group | 354 | 41.4 |
Low, moderate, and high food consumption groups consisted of 344 patients (40.4%), 152 patients (17.9%), and 354 patients (41.7%), respectively (Table 1). Of these, 63 patients, 6 patients, and 6 patients had bacteremia in the low, moderate, and high food consumption group, respectively. In order to distinguish those patients who had decreased food consumption compared to almost normal food consumption, low and moderate food consumption groups were combined and compared to the high food consumption group. Comparison of the combined low and moderate food consumption group versus the high food consumption group revealed a sensitivity of 92.0% and a negative predictive value of 98.3% for excluding true bacteremia. Conversely, the specificity (45.1%) and the positive predictive value (13.9%) were poor.
In the univariate analysis, the following variables were not statistically significantly associated with true bacteremia: age, heart rate, and leukocyte counts. Significant univariate predictors of bacteremia and their associated cut‐off points were body temperature of 36 or 38C (odds ratio [OR], 2.5; 95% CI, 1.54.4), CRP 10.0 mg/dL (OR, 2.0; 95% CI, 1.23.2), and food consumption (OR, 8.5; 95% CI, 3.818.6) (Table 2). There was no evidence of colinearity. In the final stepwise logistic regression (Table 3), the significant predictors of bacteremia were body temperature of 36 or 38C (OR, 2.4; 95% CI, 1.44.2; P = 0.002), C‐reactive protein of 10.0 mg/dL (OR, 1.9; 95% CI, 1.23.0; P = 0.011), and food consumption (OR, 7.5; 95% CI, 3.416.6; P < 0.001). We identified only 6 patients with bacteremia in the high food consumption group. Three of the patients had been previously treated with antibiotics for conditions including infective endocarditis, osteomyelitis, and myelodysplasic syndrome.
Variables | Blood Culture Result | P Value | OR (95% CI) | |
---|---|---|---|---|
Negative (n = 729) (%) | Positive (n = 75) | |||
| ||||
Age, years | 66.6 | 69.0 | ||
Mean SD | 16.9 | 13.5 | ||
70 | 408 (56.0) | 43 (57.3) | 0.7 | |
Heart rate, beats/min | 90.5 | 96.3 | ||
Mean SD | 19.0 | 20.3 | ||
90 | 368 (50.4) | 43 (57.3) | 0.3 | |
Temperature, C | 38.0 | 38.6 | ||
Mean SD | 1.0 | 1.6 | ||
36, 38 | 444 (61.0) | 61 (81.3) | <0.001 | 2.5 (1.54.4) |
Leukocyte count, cells/L | 10.1 | 11.2 | ||
Mean SD, 100 | 12.1 | 7.4 | ||
120 103, <4 103 | 336 (46.1) | 38 (50.7) | 0.4 | |
C‐reactive protein | ||||
Mean SD | 7.8 | 10.0 | ||
10.0 | 245 (33.6) | 39 (52.0) | 0.0004 | 2.0 (1.23.2) |
Food consumption | ||||
Low and moderate | 426 (58.9) | 69 (92.0) | ||
High | 350 (48.0) | 6 (8.0) | <0.001 | 8.5 (3.818.6) |
Variables | OR (95% CI) | P Value |
---|---|---|
| ||
Temperature, C 36 or 38 | 2.4 (1.44.2) | 0.002 |
C‐reactive protein, mg/dL 10.0 | 1.9 (1.23.0) | 0.011 |
Food consumption High vs low and moderate | 7.5 (3.416.6) | <0.001 |
On further analysis, we excluded patients who had received antibiotics before blood culture acquisition. There were 661 patients in this subanalysis. Low, moderate, and high food consumption groups consisted of 282 patients (41.4%), 118 patients (17.3%), and 261 patients (38.3%), respectively. Of these, 50 patients (17.7%), 5 patients (4.2%), and 4 patients (1.5%) had bacteremia in the low, moderate, and high food consumption groups, respectively. The sensitivity and negative predictive values were 93.2% and 98.5%, respectively. In the stepwise logistic regression, significant predictors of bacteremia were body temperature of 36 or 38C (OR, 3.0; 95% CI, 1.55.6; P = 0.001), CRP 10.0 mg/dL (OR, 2.1; 95% CI, 1.23.7; P = 0.006), and food consumption (OR, 9.3; 95% CI, 3.326.1; P < 0.001).
DISCUSSION
We found that in a group of 851 Japanese patients who were suspected with bacterial infection, the estimated food consumption was negatively associated, both significantly and independently, with the subsequent isolation of microorganisms from their blood cultures. If validated in other studies, this simple rule of thumb can provide the clinician with reasonable confidence that a febrile patient has a low probability of being bacteremic, as long as the appetite remains normal. Both the sensitivity and the negative predictive value were extremely high at 92.3% and 98.3%, respectively, suggesting that adequate oral intake is a strong marker against the presence of bacteremia. In this study, it was the strongest predictor of bacteremia in multivariate analysis. After including only antibiotic‐naive patients, the sensitivity and the negative predictive values were 93.2% and 98.5%, respectively. Administration of antibiotics may lead to improved appetite in febrile patients despite bacteremia in the presence of fever, and therefore, inquiring about recent or current antimicrobial usage should be a requirement when considering oral intake as an indicator of bacteremia.
Our study has limitations. Since we did not make treatment decisions based on oral intake, we cannot conclude that it is safe to withhold antibiotic treatment on the basis of food intake alone. Additionally, this study would need to be repeated across many different age groups and racial groups to ensure applicability to the general population. It is also unknown whether this rule would be applicable to patients with underlying immunosuppression. Finally, although inter‐rater reliability was high in our center, nurses in other settings may not be as diligent in their assessment of food consumption. The high inter‐assessor reliability in our setting, however, suggests that objective assessment of food intake can be performed reliably in settings in which accurate documentation of food consumption is expected.
In summary, we found that normal food intake was strongly and negatively associated with bacteremia in febrile patients. This observation, if validated in other settings, may serve as a simple aid to assist in the clinical diagnosis of bacteremia or for recruitment of patients with a high likelihood of bacteremia into clinical trials.
Acknowledgements
The authors thank Drs T. Morimoto and S. Ueda for assistance with statistical analysis, Ms M. Takigawa, and M. Kudo for collection of data, and Drs T. Oguri and Tachibana for infectious disease consultation on the pathogenicity of the microbiological organisms.
- 2001 SCCM/ESICM/ACCP/ATS/SIS International Sepsis Definitions Conference. Crit Care Med. 2003;31(4):1250–1256. , , , et al.
- Septic shock. Lancet. 2005;365(9453):63–78. , , .
- A simple index to identify occult bacterial infection in adults with acute unexplained fever. Arch Intern Med. 1987;147(4):666–671. , , , .
- Occult bacterial infection in adults with unexplained fever. Validation of a diagnostic index. Arch Intern Med. 1990;150(6):1270–1272. , , .
- Bacteremia in febrile patients. A clinical model for diagnosis. Arch Intern Med. 1991;151(9):1801–1806. , , , , .
- Blood cultures. Ann Intern Med. 1987;106(2):246–253. , .
- The clinical significance of positive blood cultures in the 1990s: a prospective comprehensive evaluation of the microbiology, epidemiology, and outcome of bacteremia and fungemia in adults. Clin Infect Dis. 1997;24(4):584–602. , , , et al.
- Effect of iodophor vs iodine tincture skin preparation on blood culture contamination rate. JAMA. 1993;269(8):1004–1006. , , .
- Predicting bacteremia in patients with sepsis syndrome. Academic Medical Center Consortium Sepsis Project Working Group. J Infect Dis. 1997;176(6):1538–1551. , , , et al.
- Clinical issues of blood cultures. Arch Intern Med. 1994;154(8):841–849. , .
- High frequency of pseudobacteremia at a university hospital. Infect Control Hosp Epidemiol. 1997;18(3):200–202. , , .
- Predicting bacteremia in hospitalized patients. A prospectively validated model. Ann Intern Med. 1990;113(7):495–500. , , , .
- Decisions made by critical care nurses during mechanical ventilation and weaning in an Australian intensive care unit. Am J Crit Care. 2007;16(5):434–443; quiz 444. , , , .
- Updated review of blood culture contamination. Clin Microbiol Rev. 2006;19(4):788–802. , .
- Minimizing the workup of blood culture contaminants: implementation and evaluation of a laboratory‐based algorithm. J Clin Microbiol. 2002;40(7):2437–2444. , , , et al.
- Evaluation of positive blood cultures. Guidelines for early differentiation of contaminated from valid positive cultures. Arch Intern Med. 1972;130(1):84–87. , .
- Predicting bacteremia at the bedside. Clin Infect Dis. 2004;38(3):357–362. , , , et al.
- Local Regression and Likelihood. New York, NY: Springer; 1999. .
- 2001 SCCM/ESICM/ACCP/ATS/SIS International Sepsis Definitions Conference. Crit Care Med. 2003;31(4):1250–1256. , , , et al.
- Septic shock. Lancet. 2005;365(9453):63–78. , , .
- A simple index to identify occult bacterial infection in adults with acute unexplained fever. Arch Intern Med. 1987;147(4):666–671. , , , .
- Occult bacterial infection in adults with unexplained fever. Validation of a diagnostic index. Arch Intern Med. 1990;150(6):1270–1272. , , .
- Bacteremia in febrile patients. A clinical model for diagnosis. Arch Intern Med. 1991;151(9):1801–1806. , , , , .
- Blood cultures. Ann Intern Med. 1987;106(2):246–253. , .
- The clinical significance of positive blood cultures in the 1990s: a prospective comprehensive evaluation of the microbiology, epidemiology, and outcome of bacteremia and fungemia in adults. Clin Infect Dis. 1997;24(4):584–602. , , , et al.
- Effect of iodophor vs iodine tincture skin preparation on blood culture contamination rate. JAMA. 1993;269(8):1004–1006. , , .
- Predicting bacteremia in patients with sepsis syndrome. Academic Medical Center Consortium Sepsis Project Working Group. J Infect Dis. 1997;176(6):1538–1551. , , , et al.
- Clinical issues of blood cultures. Arch Intern Med. 1994;154(8):841–849. , .
- High frequency of pseudobacteremia at a university hospital. Infect Control Hosp Epidemiol. 1997;18(3):200–202. , , .
- Predicting bacteremia in hospitalized patients. A prospectively validated model. Ann Intern Med. 1990;113(7):495–500. , , , .
- Decisions made by critical care nurses during mechanical ventilation and weaning in an Australian intensive care unit. Am J Crit Care. 2007;16(5):434–443; quiz 444. , , , .
- Updated review of blood culture contamination. Clin Microbiol Rev. 2006;19(4):788–802. , .
- Minimizing the workup of blood culture contaminants: implementation and evaluation of a laboratory‐based algorithm. J Clin Microbiol. 2002;40(7):2437–2444. , , , et al.
- Evaluation of positive blood cultures. Guidelines for early differentiation of contaminated from valid positive cultures. Arch Intern Med. 1972;130(1):84–87. , .
- Predicting bacteremia at the bedside. Clin Infect Dis. 2004;38(3):357–362. , , , et al.
- Local Regression and Likelihood. New York, NY: Springer; 1999. .
Copyright © 2012 Society of Hospital Medicine